The Jasper Blog
Resources for AI in Marketing

Reflections from Cannes: Why Brand and People Still Matter Most in the Era of AI
At Cannes Lions 2025, one truth stood out: brand, trust, and authenticity are making a powerful comeback.
June 24, 2025
|
Loreal Lynch
June
17
|
Megan Dubin
Interactive Tool: Benchmark Your AI in Marketing Strategy
Discover your marketing team's AI maturity. Benchmark your strategy, compare with peers, and uncover gaps with an interactive tool.
June
10
|
Timothy Young
Introducing the New Jasper: The First Multi-Agent Platform Built for Marketers
Discover the new Jasper AI experience, built for enterprise marketers to scale content and strategy with precision and true-to-brand authenticity.
June
10
|
Megan Dubin
Why the Traditional Content Lifecycle No Longer Works
Learn why traditional content workflows break down, and how AI can rewire the marketing lifecycle to unlock scale, consistency, and ROI.
All posts
Join the official Jasper community
Meet marketers, creators, & more sharing tips for generating amazing content 10X faster using AI.

Scaling AI for the Enterprise: How Marketing Leaders Drive Impact
High-performing marketing teams are scaling AI with structure and strategy. Learn how CMOs can lead the way to drive organization-wide impact.
July 1, 2025
|
Megan Dubin
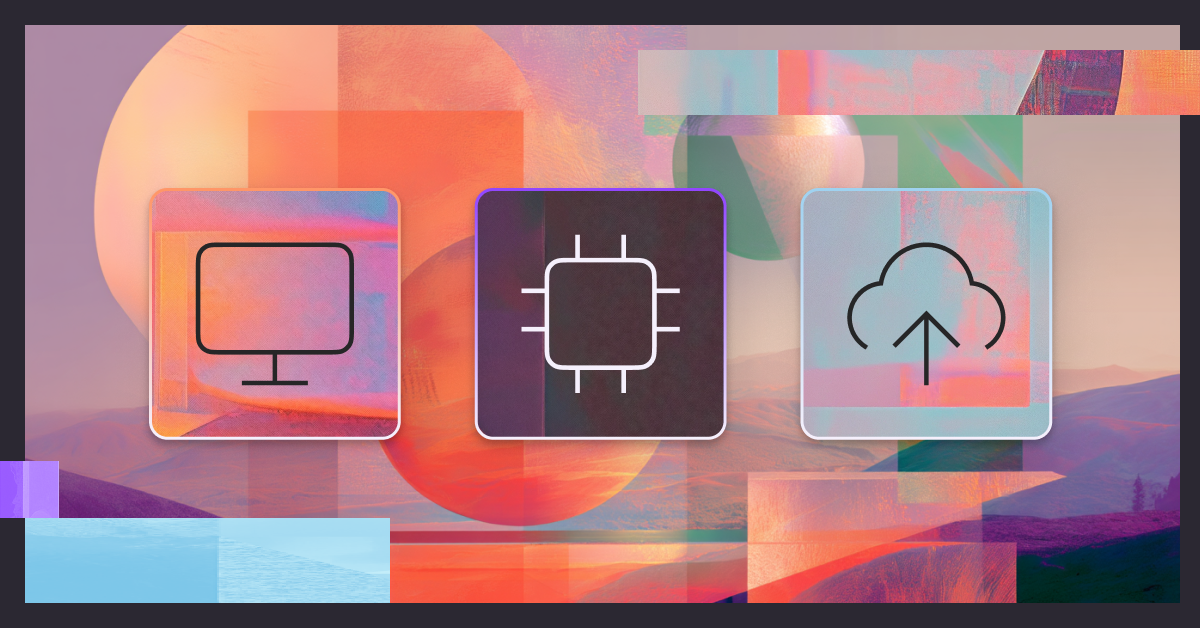
Interactive Tool: Benchmark Your AI in Marketing Strategy
Discover your marketing team's AI maturity. Benchmark your strategy, compare with peers, and uncover gaps with an interactive tool.
June 17, 2025
|
Megan Dubin
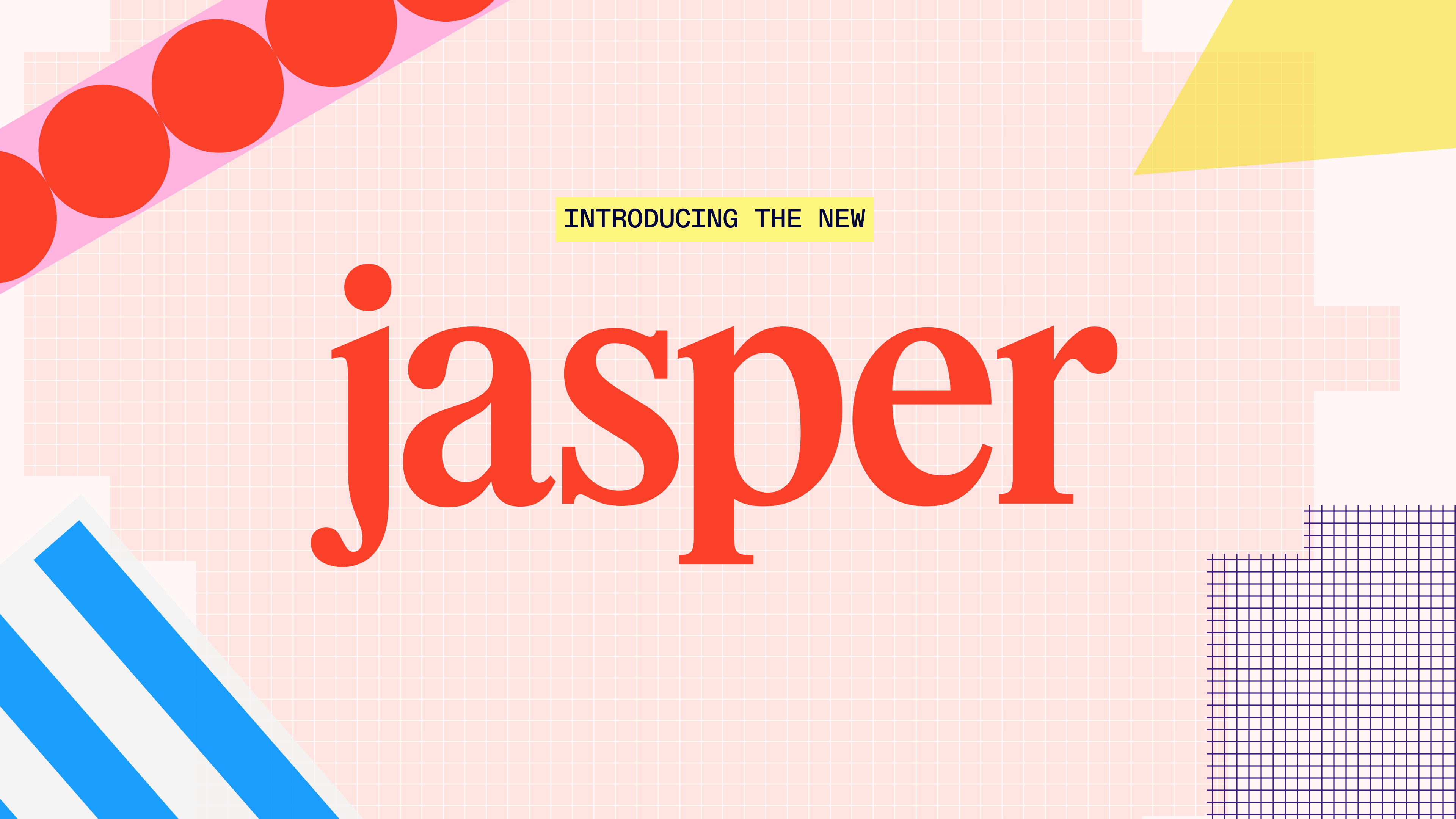
Introducing the New Jasper: The First Multi-Agent Platform Built for Marketers
Discover the new Jasper AI experience, built for enterprise marketers to scale content and strategy with precision and true-to-brand authenticity.
June 10, 2025
|
Timothy Young

Why the Traditional Content Lifecycle No Longer Works
Learn why traditional content workflows break down, and how AI can rewire the marketing lifecycle to unlock scale, consistency, and ROI.
June 10, 2025
|
Megan Dubin
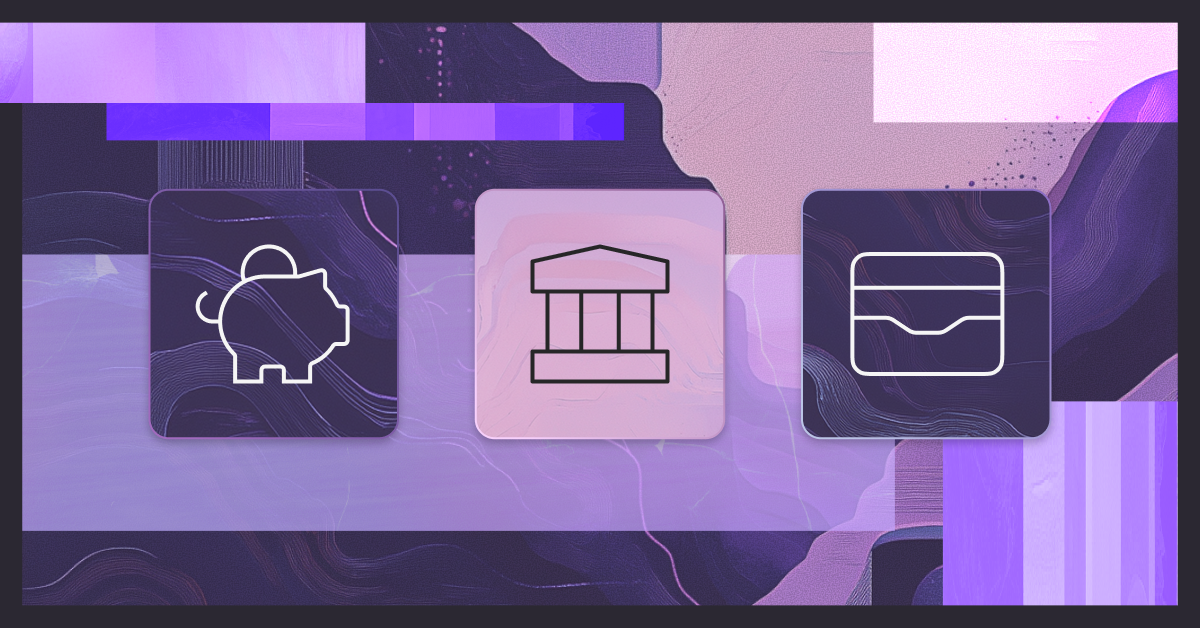
The State of AI in Financial Services Marketing 2025
Financial services marketing teams are ahead in AI maturity. Learn how they can scale responsibly and innovatively to maintain their competitive edge.
June 3, 2025
|
Jasper Marketing
.png)
How AI Agents Are Transforming Marketing Workflows
AI agents move beyond task automation to deliver real-time optimization, brand governance, and marketing outcomes across channels.
June 1, 2025
|
Jasper Marketing

The State of AI in Life Sciences Marketing 2025
Learn where life sciences marketing measures up in AI adoption and how domain-specific tools can help teams drive results.
May 29, 2025
|
Jasper Marketing
.png)
Latent Bridge Matching: Jasper AI’s Game-Changing Approach to Image Translation
Discover how latent bridge matching, pioneered by the Jasper research team, transforms image-to-image translation with unmatched speed, quality, and efficiency.
May 28, 2025
|
Jasper
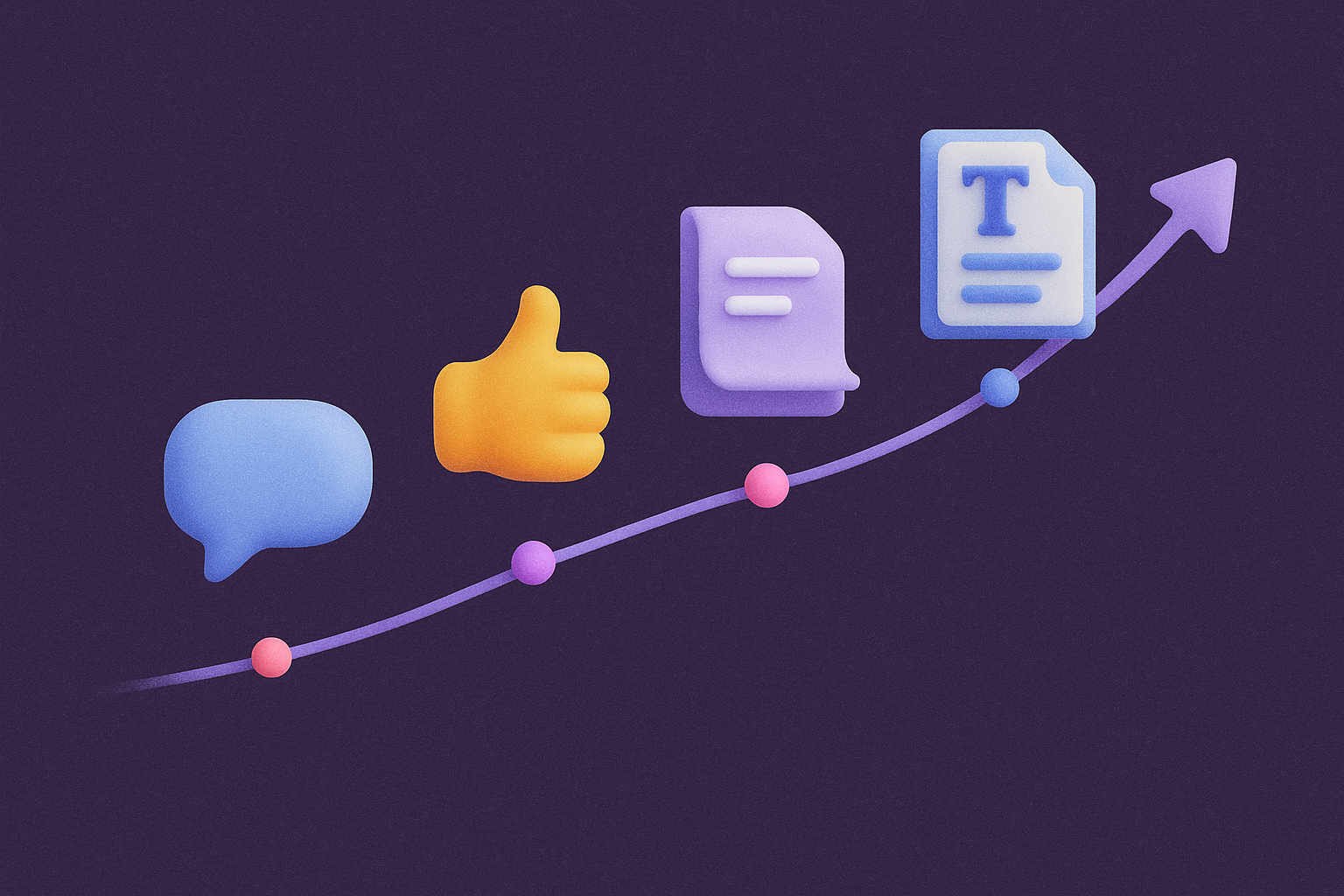
Why Domain-Specific AI is Key to Increasing Content ROI
Discover how marketers can use purpose-built AI (including an example with Jasper) to create high-quality, on-brand content at scale.
May 21, 2025
|
Tom Newton
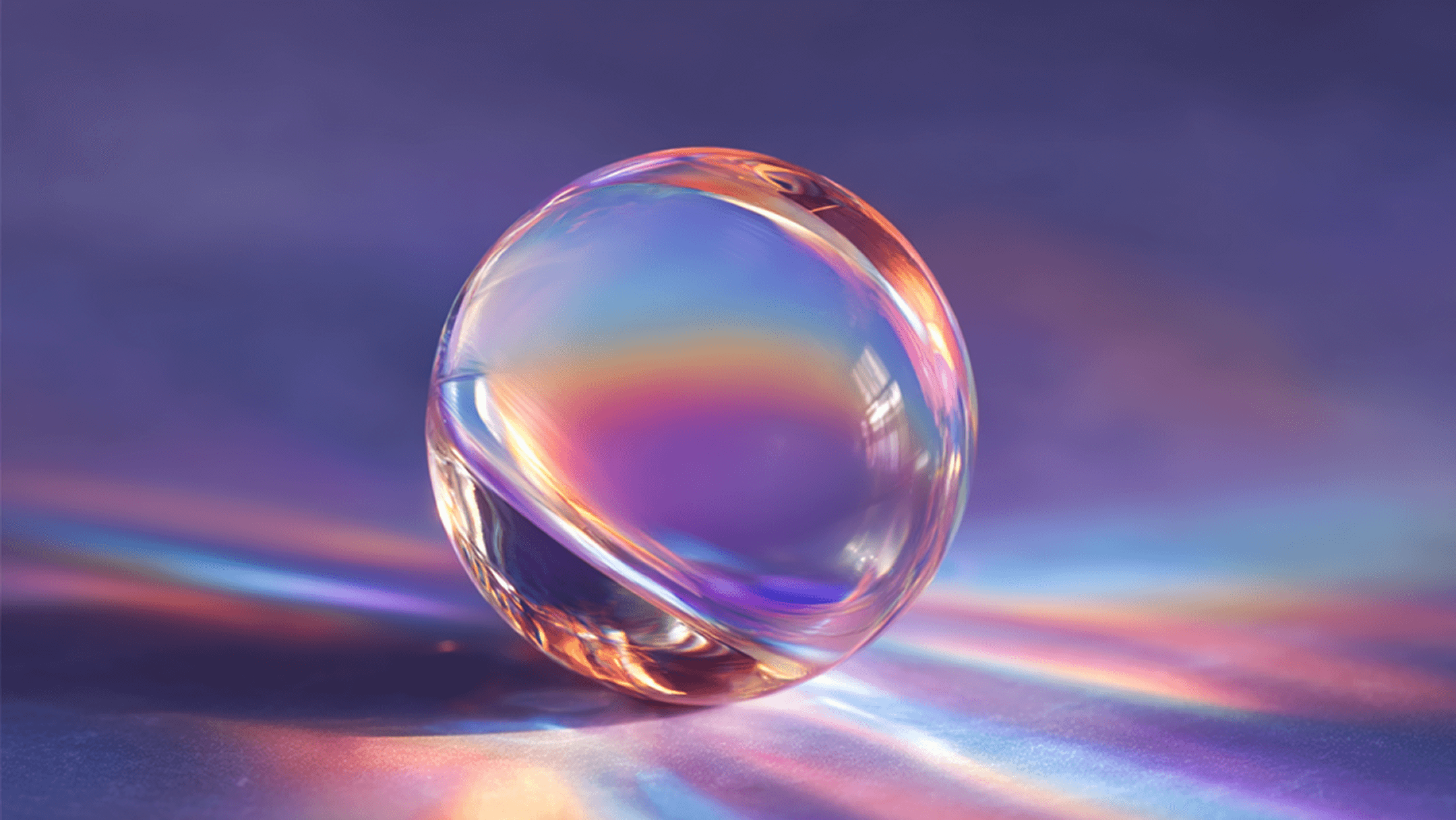
Why Context is the Ultimate Differentiator for AI in Marketing
Context is the key to making AI work in marketing. Here’s why generic tools fall short and contextual AI drives better results.
May 20, 2025
|
Loreal Lynch
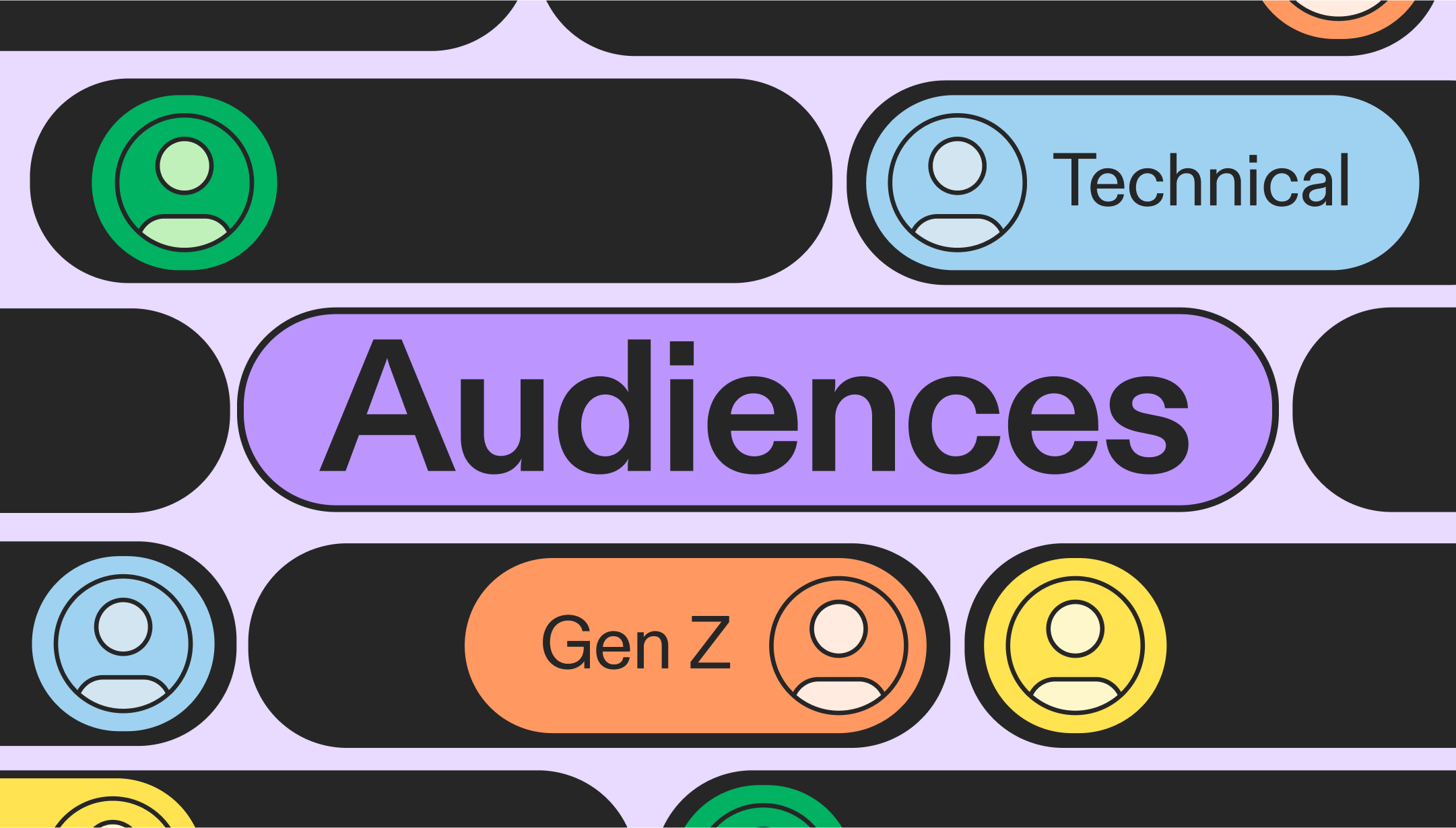
Introducing Audiences: Unlocking Campaigns That Truly Resonate
Discover how Audiences can transform marketing campaigns with dynamic, actionable audience insights.
May 14, 2025
|
Bryan Tsao
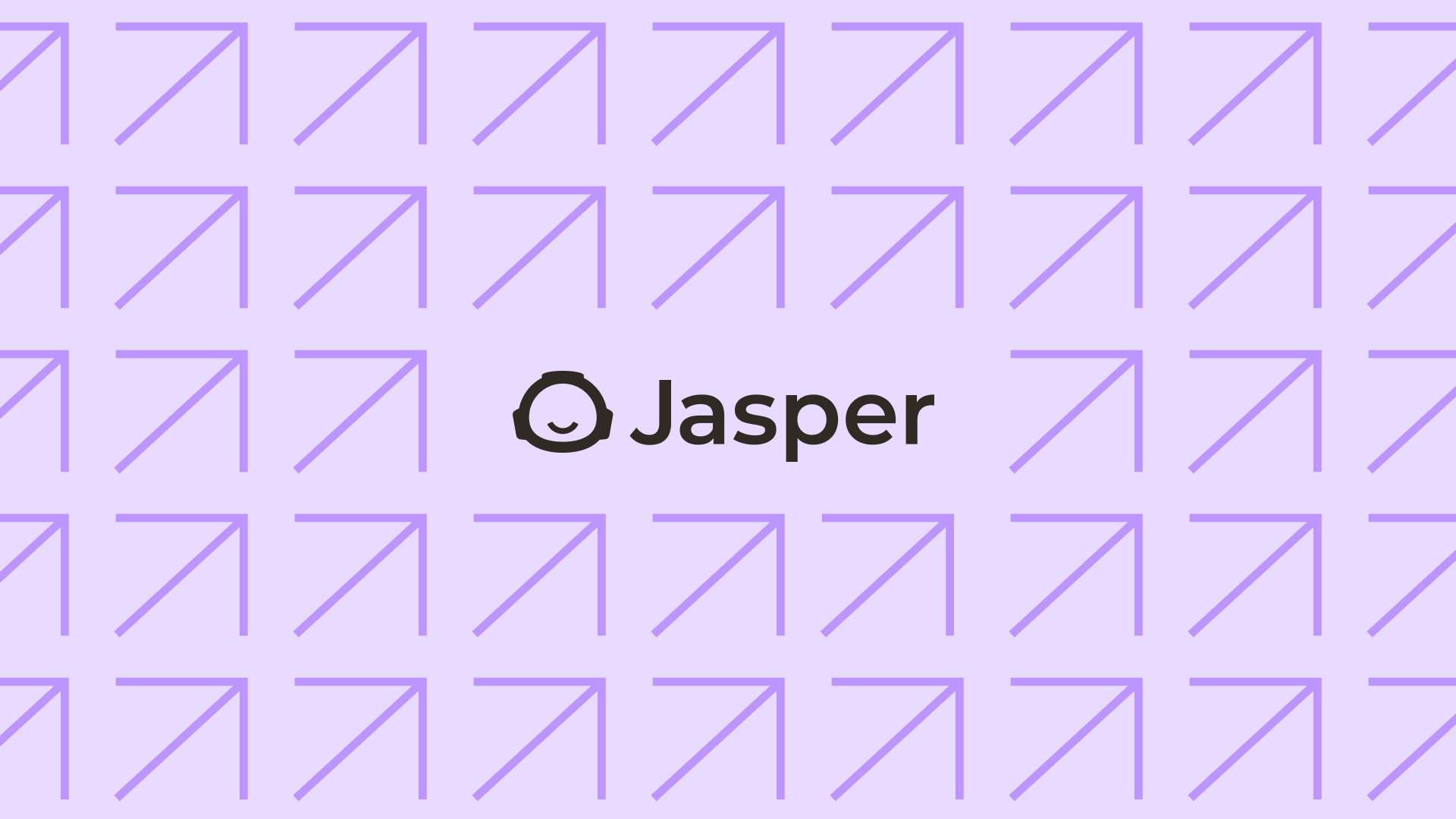
Jasper Joins NYSE LaunchPad and Launches Inaugural Customer Advisory Board
Discover how Jasper’s CAB and inclusion in the NYSE LaunchPad program are driving innovation for enterprise marketers.
May 7, 2025
|
Jasper

AI Trends Shaping Tech Marketing in 2025
Technology is the clear industry leader in AI adoption, but surprising gaps still remain to reach AI’s full potential.
April 29, 2025
|
Jasper Marketing
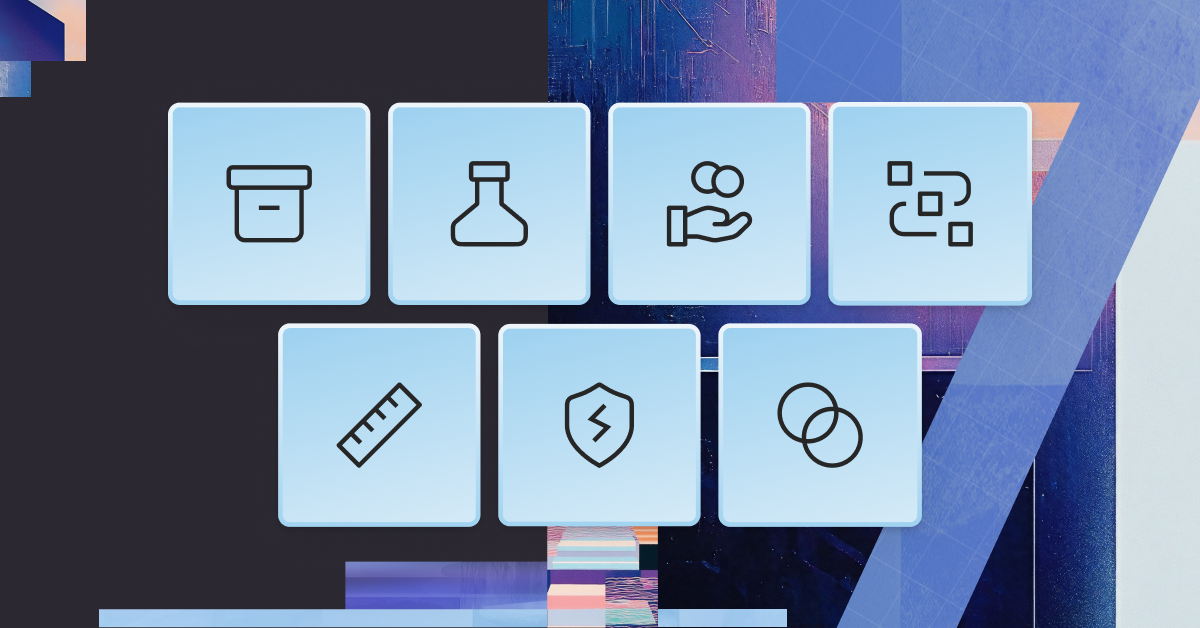
The 7 Traits of Marketing Organizations Leading in AI Maturity
Discover the 7 traits of marketing teams leading in AI—plus a roadmap to help your team unlock its full potential.
April 20, 2025
|
Jasper Marketing
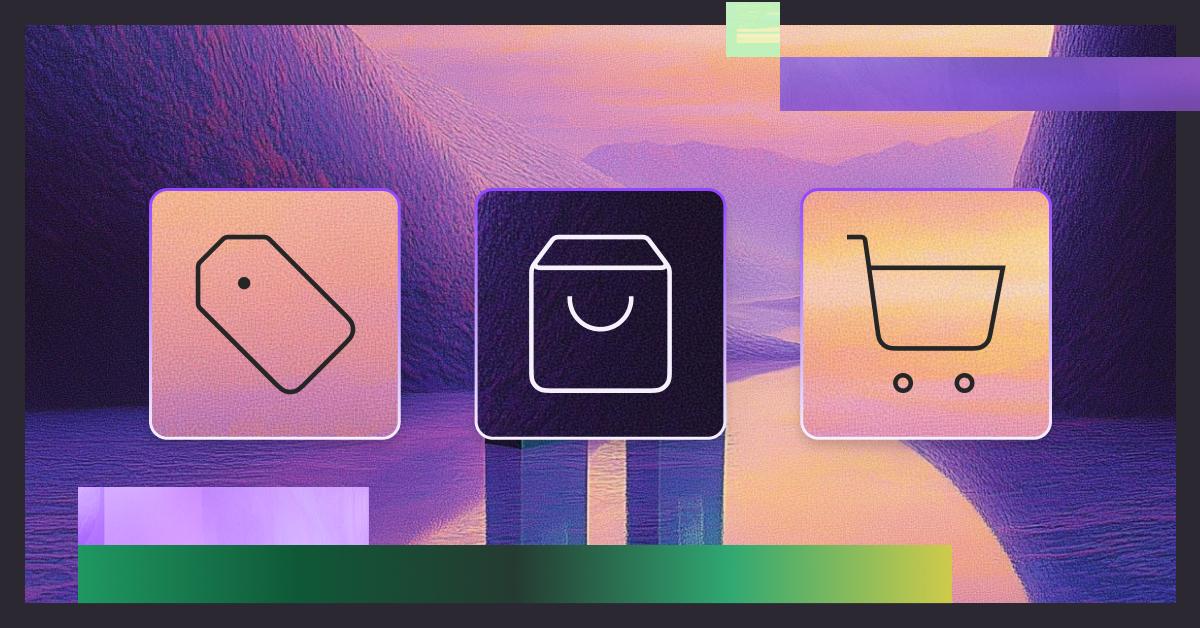
The State of AI in Retail Marketing 2025
Retail is ahead of the AI adoption curve in 2025, but gaps and opportunities remain. Get exclusive insights for retailers from Jasper’s latest research.
April 8, 2025
|
Jasper Marketing

The HERDS Framework: Mobilizing Change Leaders to Scale AI in Marketing
Learn how to drive adoption, engage stakeholders, and overcome resistance with this AI change management framework.
March 30, 2025
|
Jessica Hreha
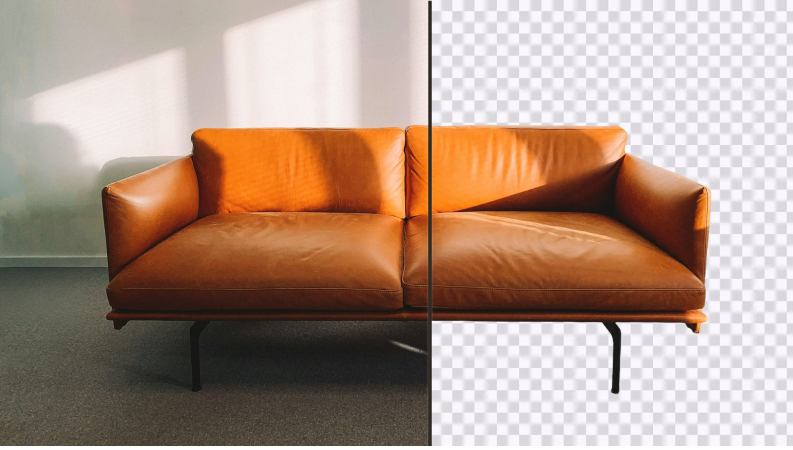
The Future of Retail Visuals with AI: A Conversation with Wayfair’s Bryan Godwin
Wayfair’s Director of AI & Visual Media on how AI is transforming retail visual content—enhancing personalization, brand consistency, and efficiency at scale
February 6, 2025
|
Loreal Lynch
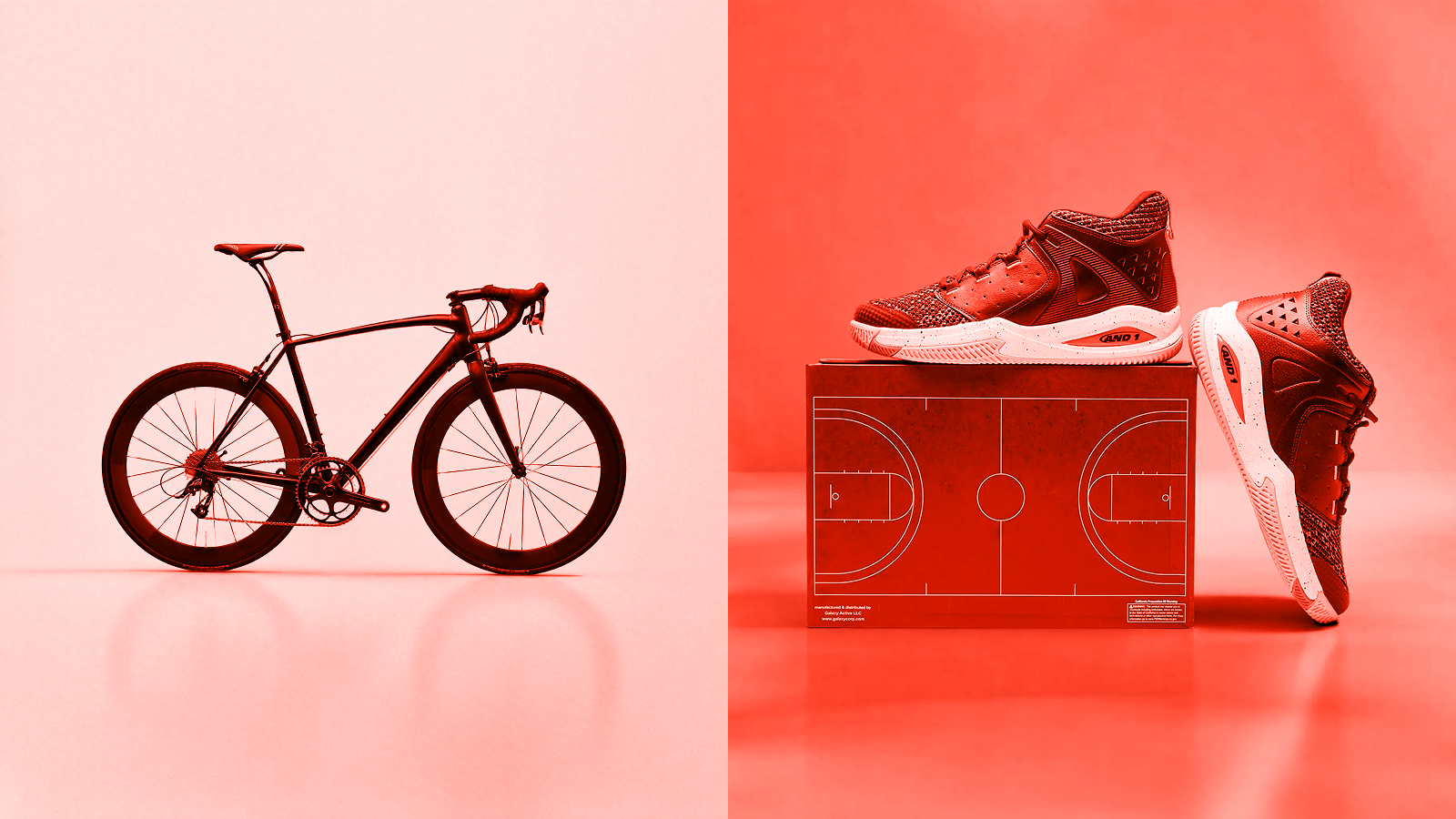
AI, Light, and Shadow: Jasper's New Research Powers More Realistic Imagery
Jasper Research Lab’s new shadow generation research and model enable brands to create more photorealistic images with enhanced realism and depth.
February 4, 2025
|
Onur Tasar

How to Redefine Your ABM Playbook Using AI
Explore key takeaways from our recent webinar on how AI can transform ABM strategies with workflow automation, personalization at scale, and more.
January 6, 2025
|
Jasper Marketing
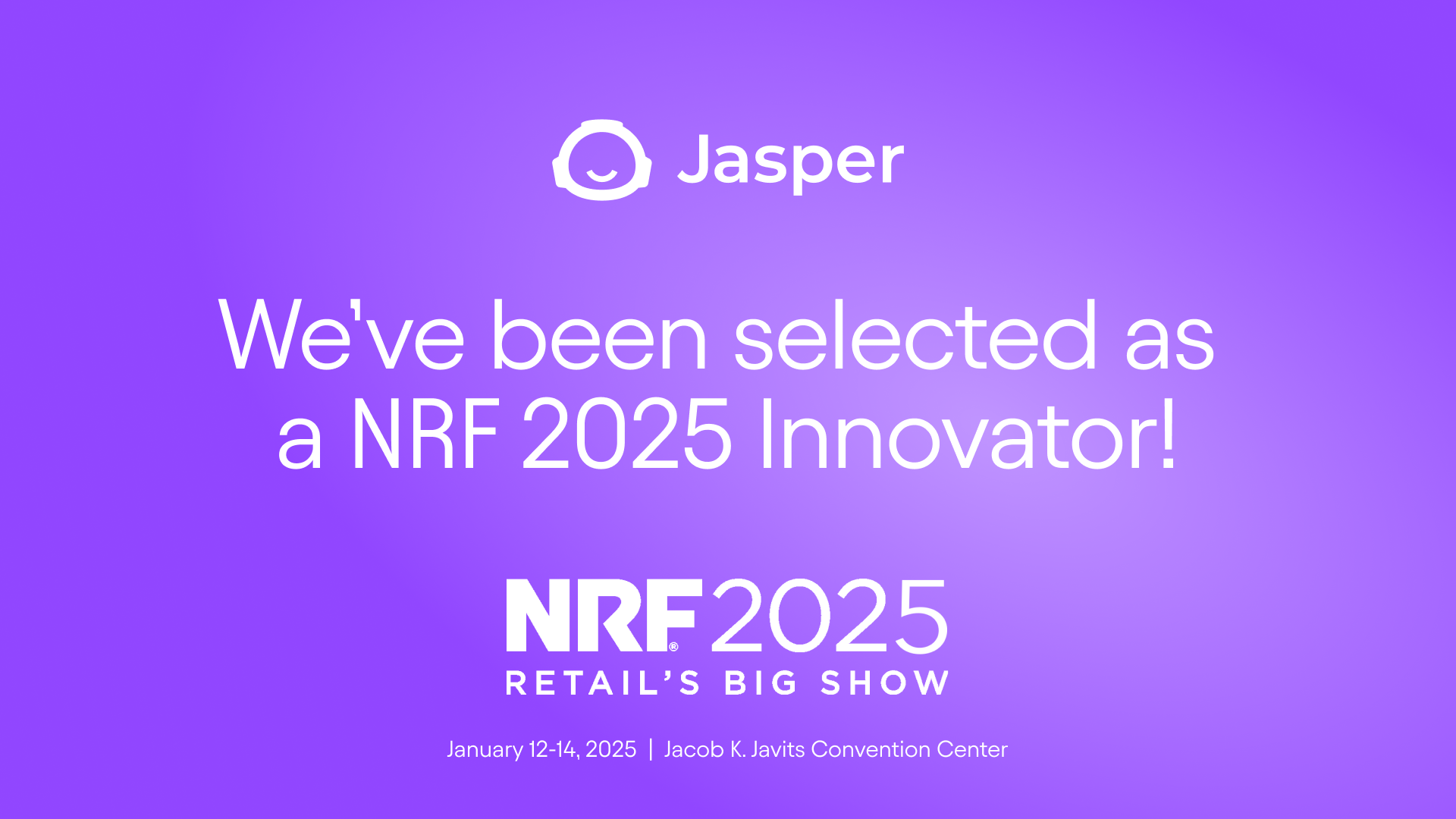
Jasper Named a 2025 NRF Innovator at Retail’s Big Show
The Innovators Showcase at NRF 2025: Retail’s Big Show recognizes the top 50 tech leaders shaping the future of retail.
December 11, 2024
|
Jasper Marketing
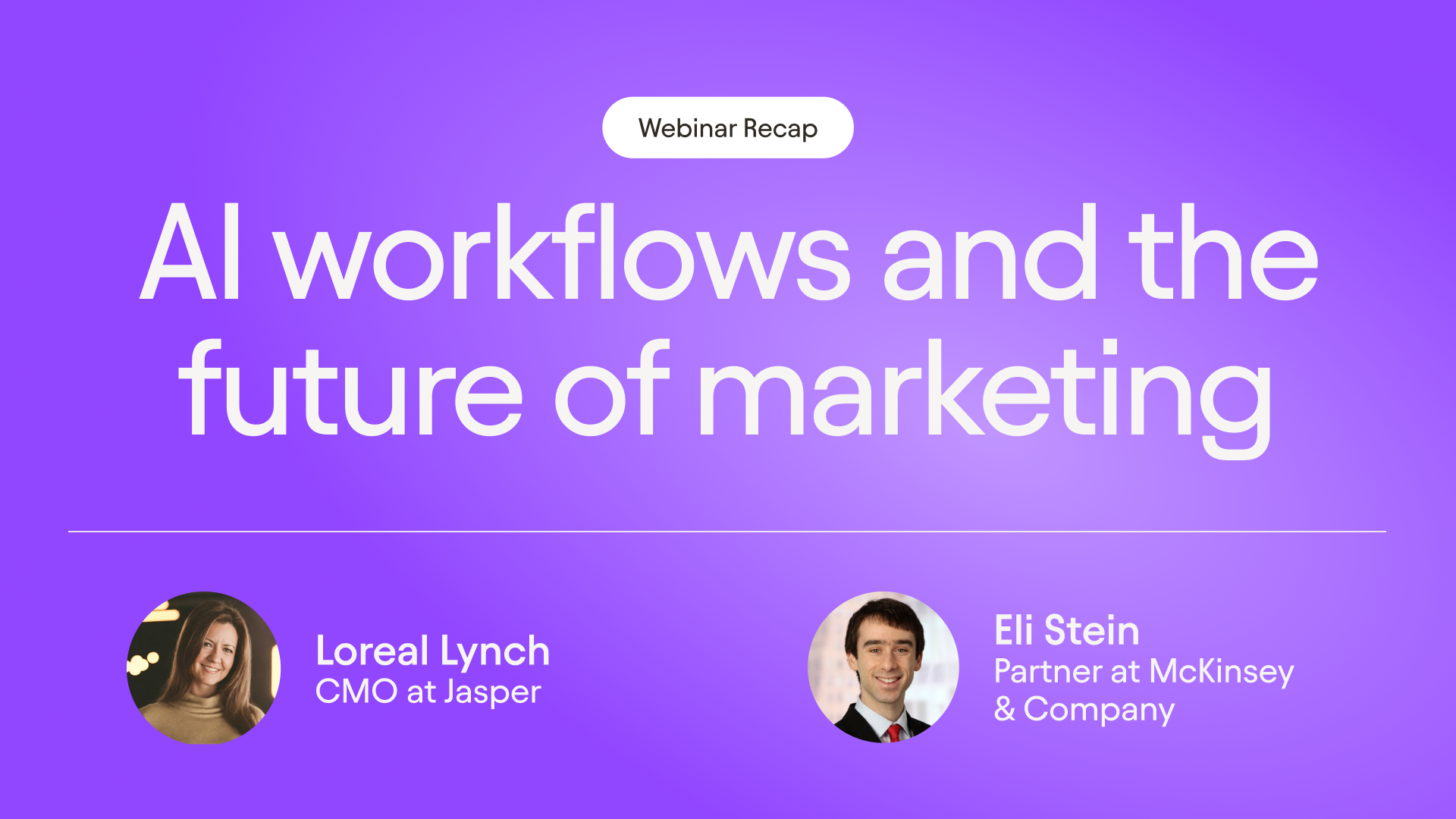
How AI Workflows are Redefining Enterprise Marketing - Insights from McKinsey and Jasper
Discover AI's impact on enterprise marketing. Learn about transformation stages, AI workflows, and scaling strategies for growth and ROI.
December 3, 2024
|
Jasper Marketing

How to Automate Your Workflows With AI
AI workflow automation is becoming one of the main levers organizations can modernize their operations. Here's how.
November 24, 2024
|
Jasper Marketing

AI workflows: What Are They And How Can Marketers Use Them?
What are AI workflows, why do they matter, and how can you accelerate key marketing processes?
November 23, 2024
|
Jasper Marketing
.png)
How To Remove the Background From a Picture
Let’s dive into the how and why of image background removal.
November 21, 2024
|
Jasper Marketing

The 2025 Guide to Using AI for Retail Marketing Strategy
Learn how AI can boost efficiency on your retail marketing efforts in 2025 and beyond.
November 19, 2024
|
Jasper Marketing

Ultimate Guide To Marketing For Retail
AI is meaningfully reshaping retail, from more efficient marketing campaigns to inventory prediction and beyond.
November 14, 2024
|
Jasper Marketing

Best AI Use Cases For Retail Marketers
Retailers are finding innovative ways to navigate a complex landscape and tap into AI's potential in retail.
November 13, 2024
|
Jasper Marketing

How to Upscale an Image + Best Tools for 2025
Discover the tools that can help you upscale an image, remove noise, and recover intricate details.
November 12, 2024
|
Jasper Marketing
.png)
Unlocking a New Era of Retail Marketing with Jasper
Find out how Jasper is helping marketers use AI to transform retail marketing as we know it.
November 10, 2024
|
Jasper Marketing
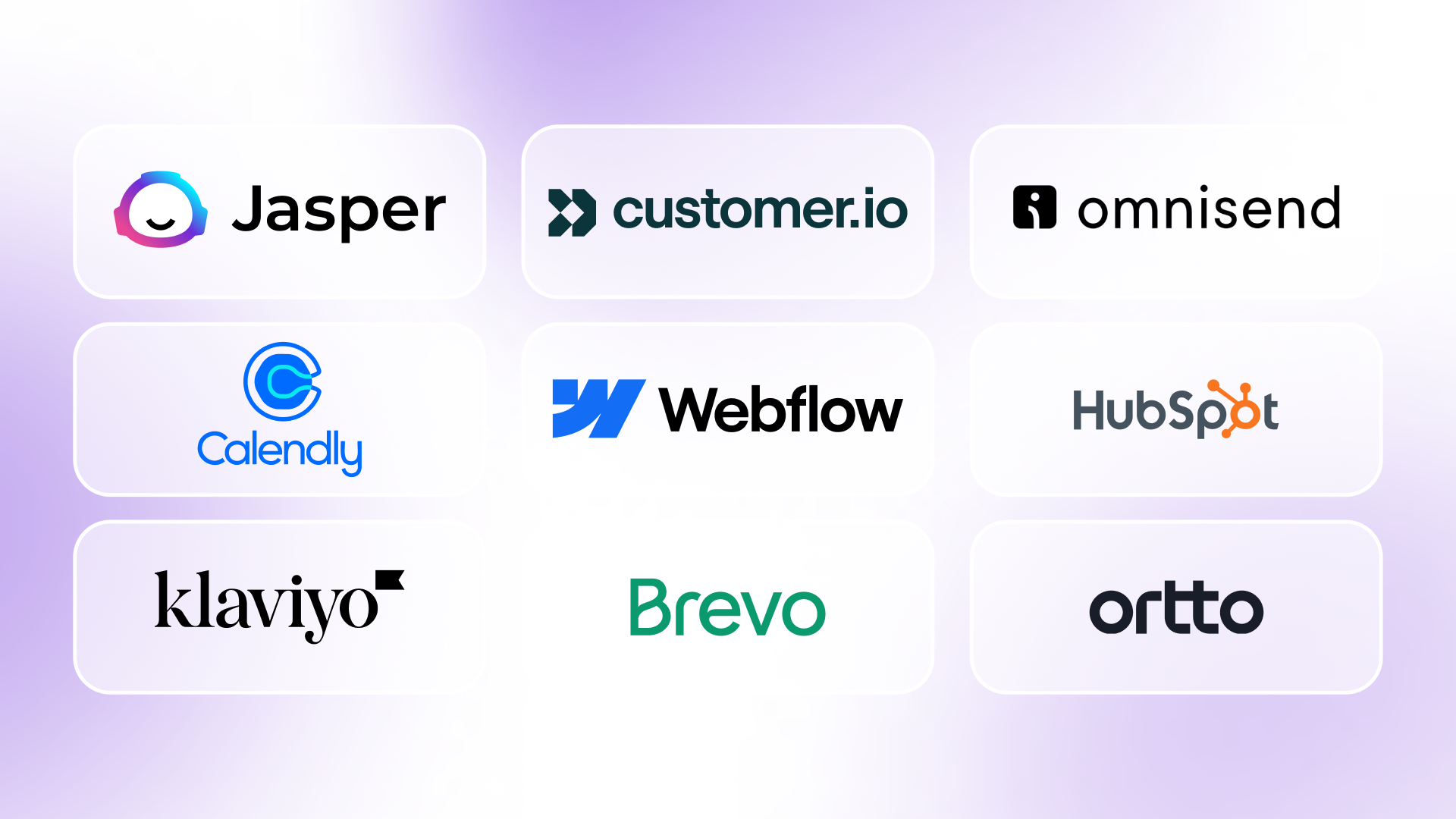
The Top 10 Marketing Tools to Build Your Tech Stack in 2025
Discover the top 10 marketing tools to build your tech stack in 2025—from content marketing and SEO to automation, social media management, and analytics tools.
November 7, 2024
|
Jasper Marketing

Best AI Tools for Business
Overwhelmed by manual tasks and data overload? Streamline your business and boost revenue with the best AI tools for business.
November 4, 2024
|
Jasper
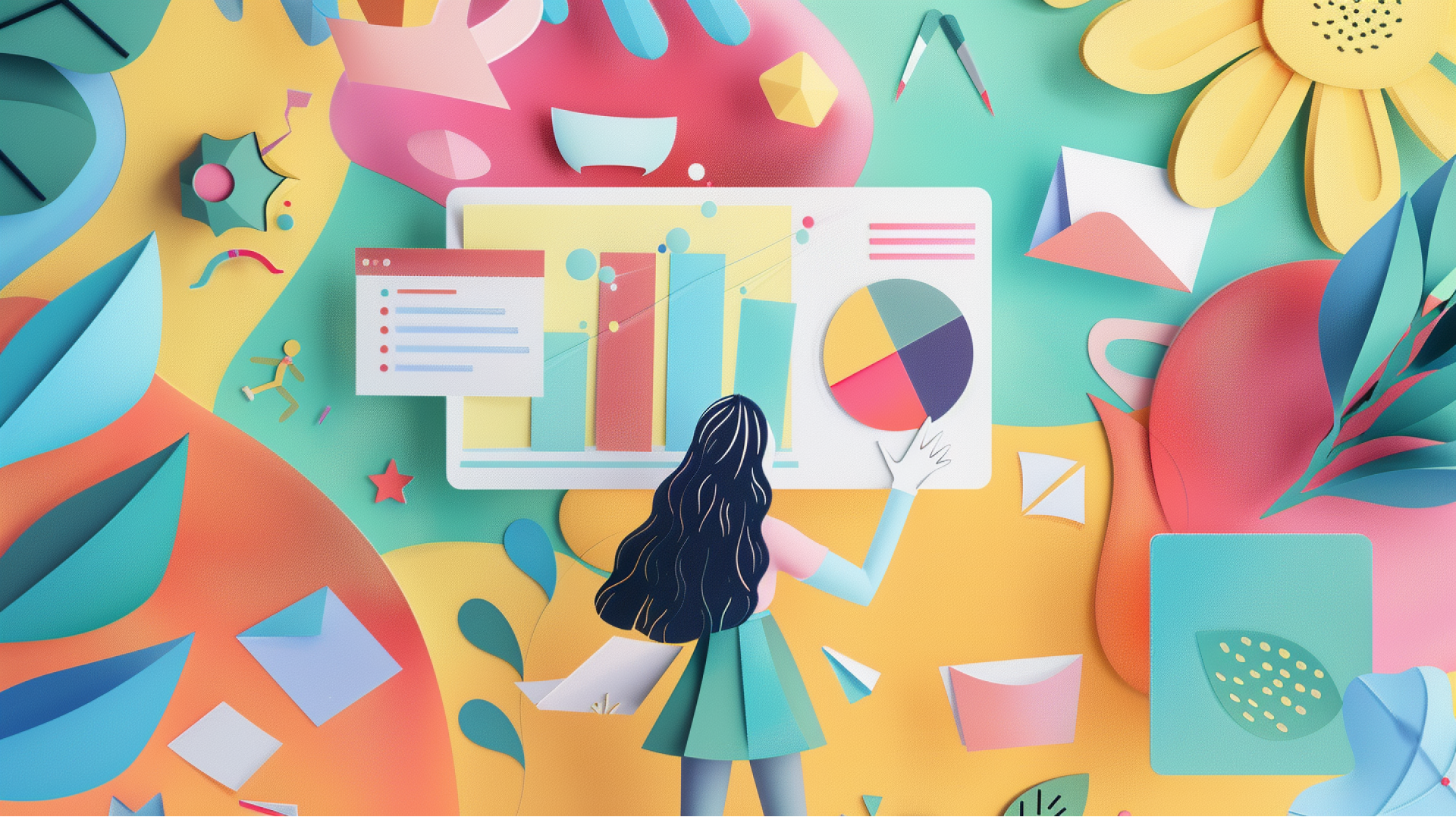
Enterprise Marketing Automation: The Ultimate Guide
Enterprise marketing automation tools help scale your business sustainably and let you overcome bottlenecks and pain points that come with scaling operations.
October 31, 2024
|
Jasper
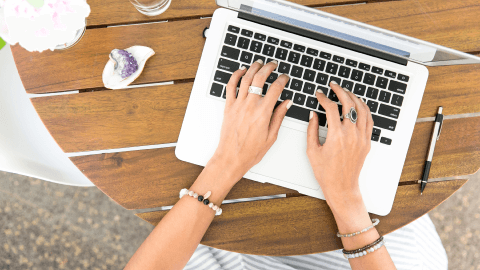
How Much Do Freelance Writers Make? [2025 Update]
Freelance writing is more profitable than ever, but how much do freelance writers make? We do a deep dive to bring you the answer to that question.
October 30, 2024
|
Jasper Marketing

What is ChatGPT: Real Life Applications, Limitations, and Alternatives
Understanding ChatGPT unlocks a wide range of potential use cases, from marketing automation to customer support. Here’s everything you need to know.
October 30, 2024
|
Jasper Marketing

How To Use Zapier: A Beginner's Guide To Workflow Automation
This beginner's guide, including examples and step-by-step instructions, teaches you how to use Zapier to automate repetitive tasks between your favorite apps.
October 20, 2024
|
Jasper Marketing
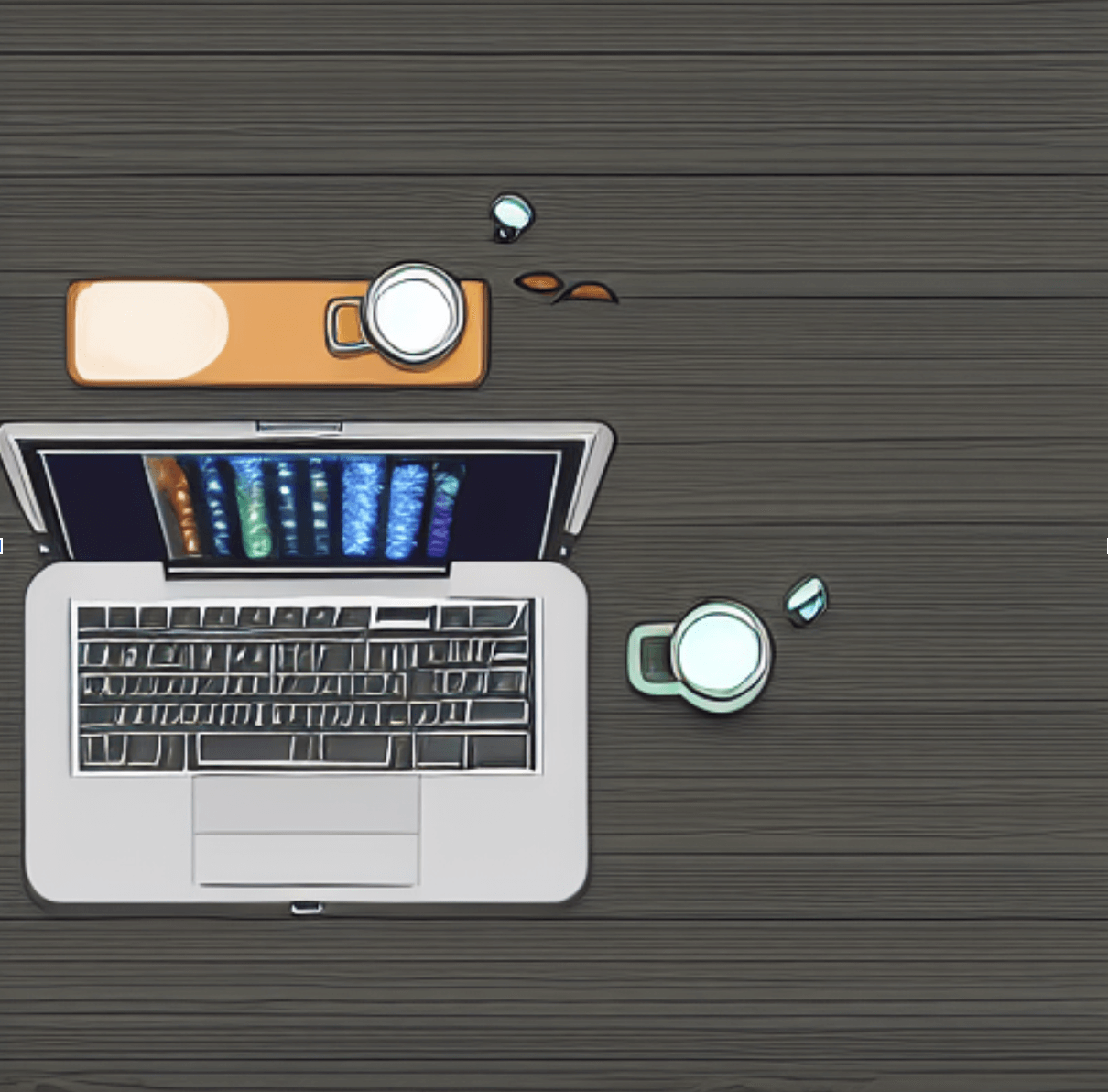
10 Best Email Subject Line Generators For 2025
Struggling to come up with interesting email subject lines for your next campaign? Use one of these email subject line generators to skyrocket your open rates.
October 15, 2024
|
Krista Doyle
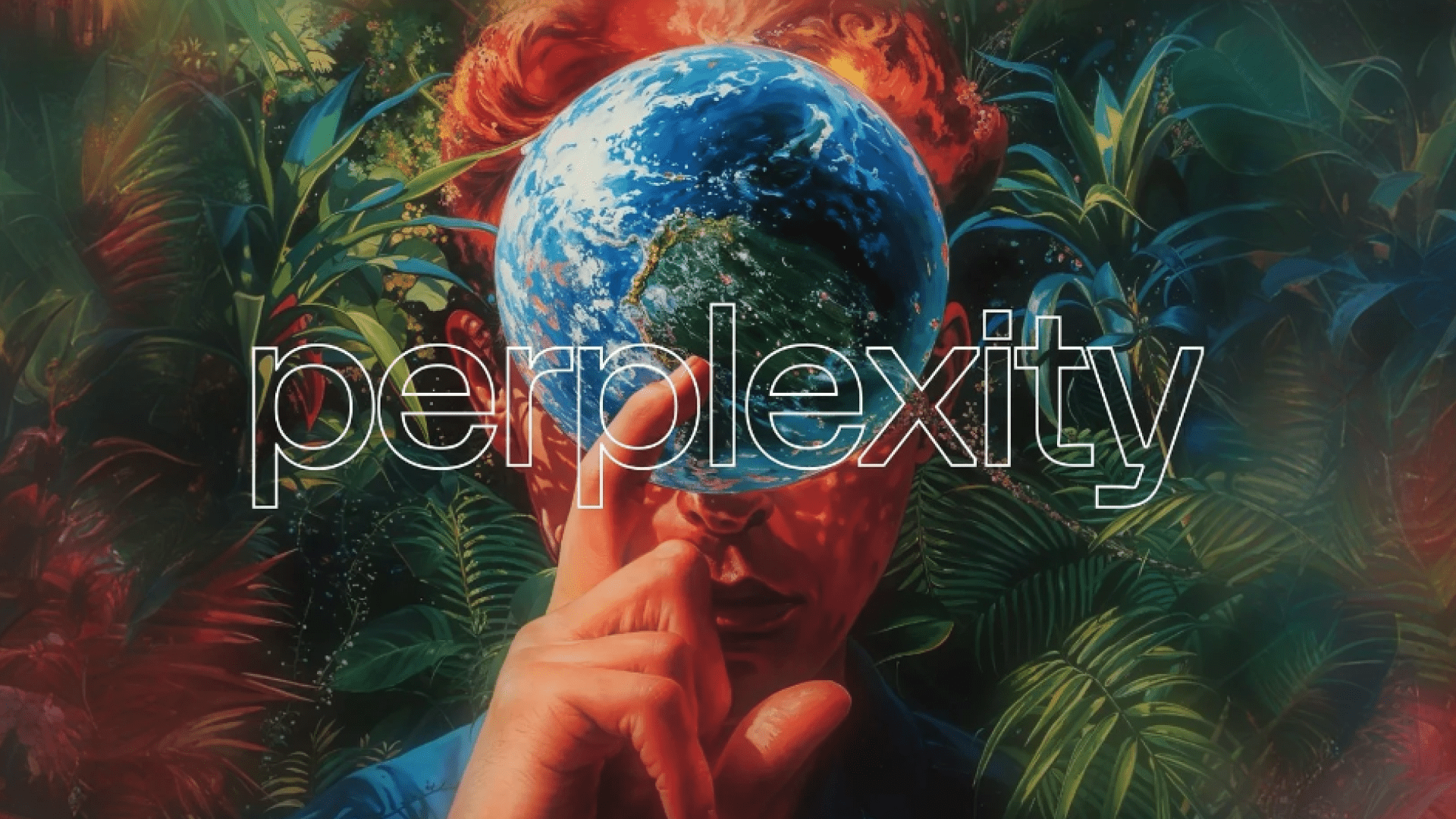
What Is Perplexity AI? Understanding One Of Google’s Biggest Search Engine Competitors
What is Perplexity AI? Is it an over-hyped replacement for Google as a search engine, or do the LLM + search features already have real-world use cases?
October 15, 2024
|
Jasper Marketing

SEO & Content Marketing: Best SEO Tools, Tips, & Best Practices [2025]
SEO should be a key part of your content marketing strategy if your goal is growth and revenue. Here are our best tips & tools to help you create SEO content.
October 14, 2024
|
Krista Doyle
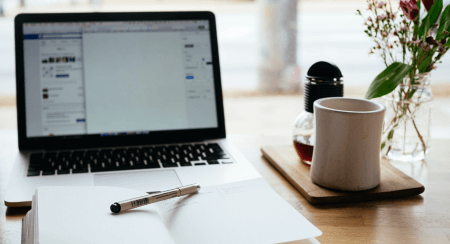
The Top 19 Content Marketing Tools You'll Need In 2025
Want to know which are the best content tools for your business? We share the top content marketing tools you need to get the most out of your content.
October 13, 2024
|
Krista Doyle
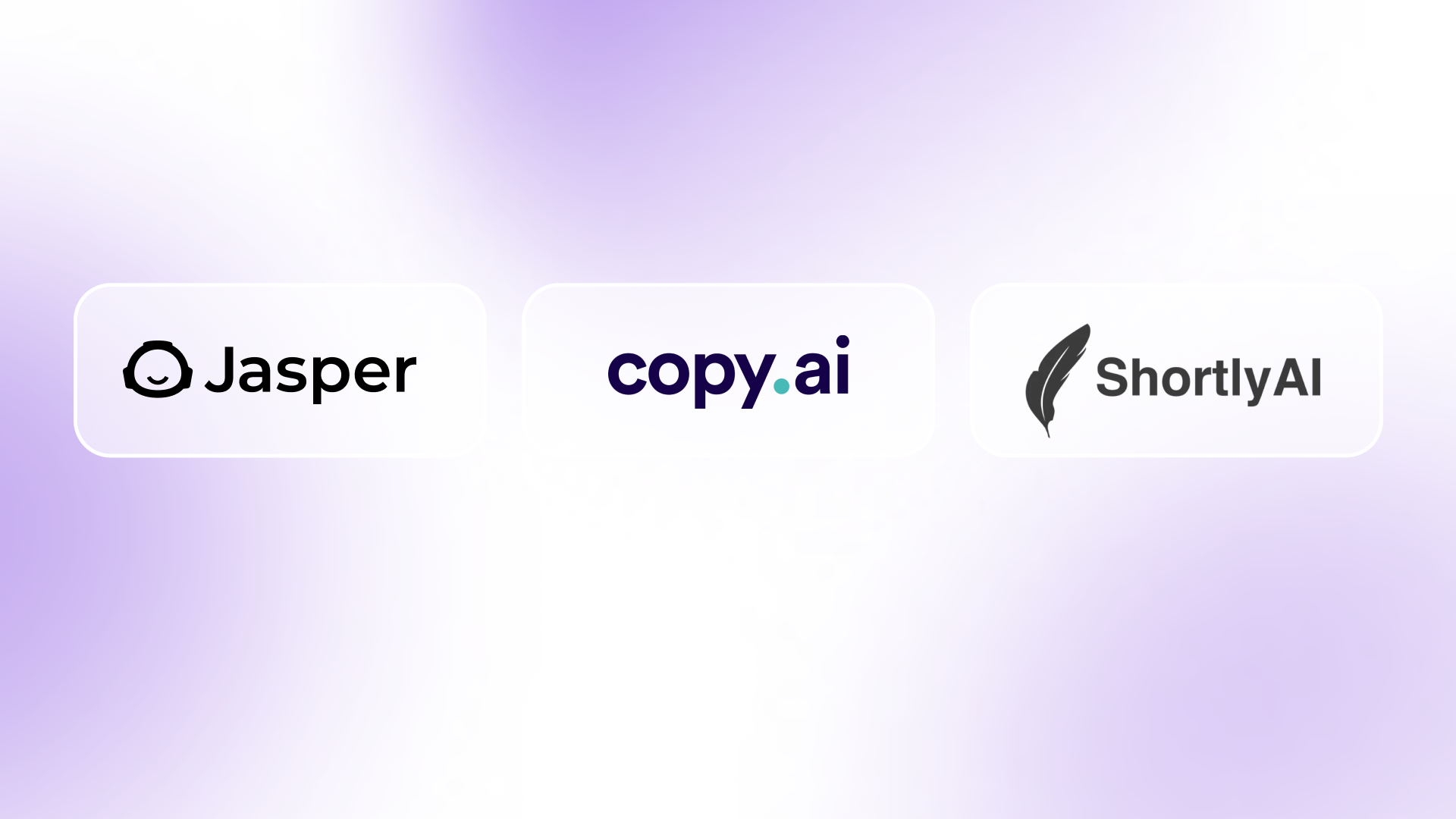
Top Writesonic Alternatives For Content Marketing, GTM, & AI-Powered Writing
Are you looking for Writesonic alternatives? Here are five of the best tools that can go toe-to-toe with Writesonic. Let’s explore the pros and cons of each.
October 13, 2024
|
Jasper Marketing
.png)
Jasper Research Unlocks New Ways to Generate On-Brand Images at Scale
Jasper Research advances AI image generation for consistent on-brand images in enterprise marketing, boosting campaign impact and efficiency.
October 13, 2024
|
Melody Meckfessel

9 Best AI Content Generators for Every Kind of Content in 2024
AI content generators are great for beating writers block and creating content that converts. Here are 9 of the best tools out there.
October 11, 2024
|
Krista Doyle

The Best 6 AI Article Rewriter Tools for 2024
Jasper's AI article rewriter tools are the best in the game. Find out why, plus see which 5 other tools we'd recommend.
October 11, 2024
|
Jasper Marketing

The 10 Best AI Video Editors to Transform Your Content
We tried ten of the best AI video editors to help you find which one fits your needs best. Here’s what we learned about their features and limitations.
October 11, 2024
|
Jasper

The Best AI Writers to Try in 2024 (and How to Use Them)
Need help creating content for your website or business? It may be time to try an AI writer. Here are the top 8, their unique features, pros and cons.
October 10, 2024
|
Krista Doyle
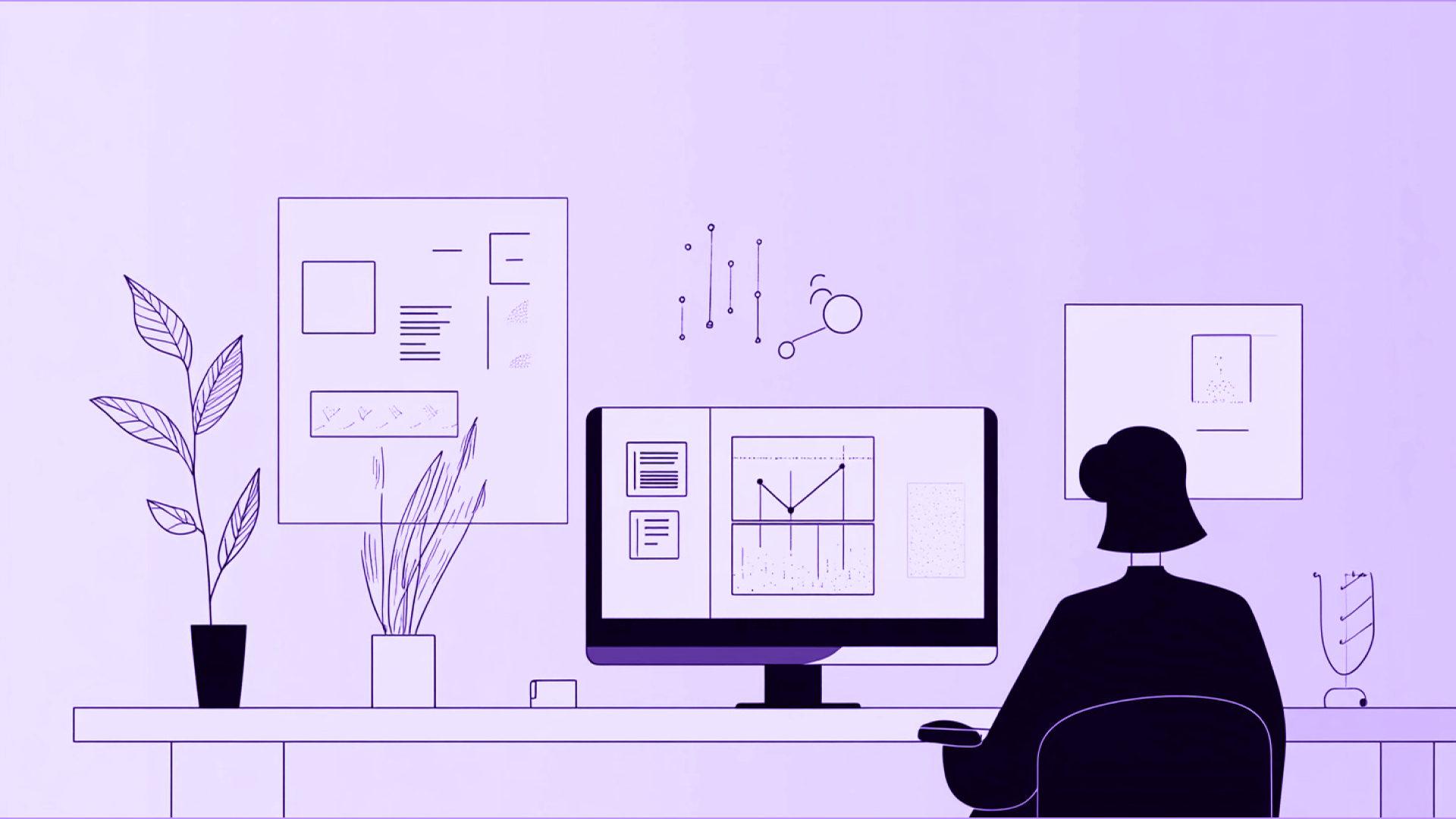
How Does Generative AI Work: The Secret To Refining Your AI Output
Find out how generative AI learns from data sets to help you optimize use cases for specific niches and needs.
October 10, 2024
|
Jasper Marketing
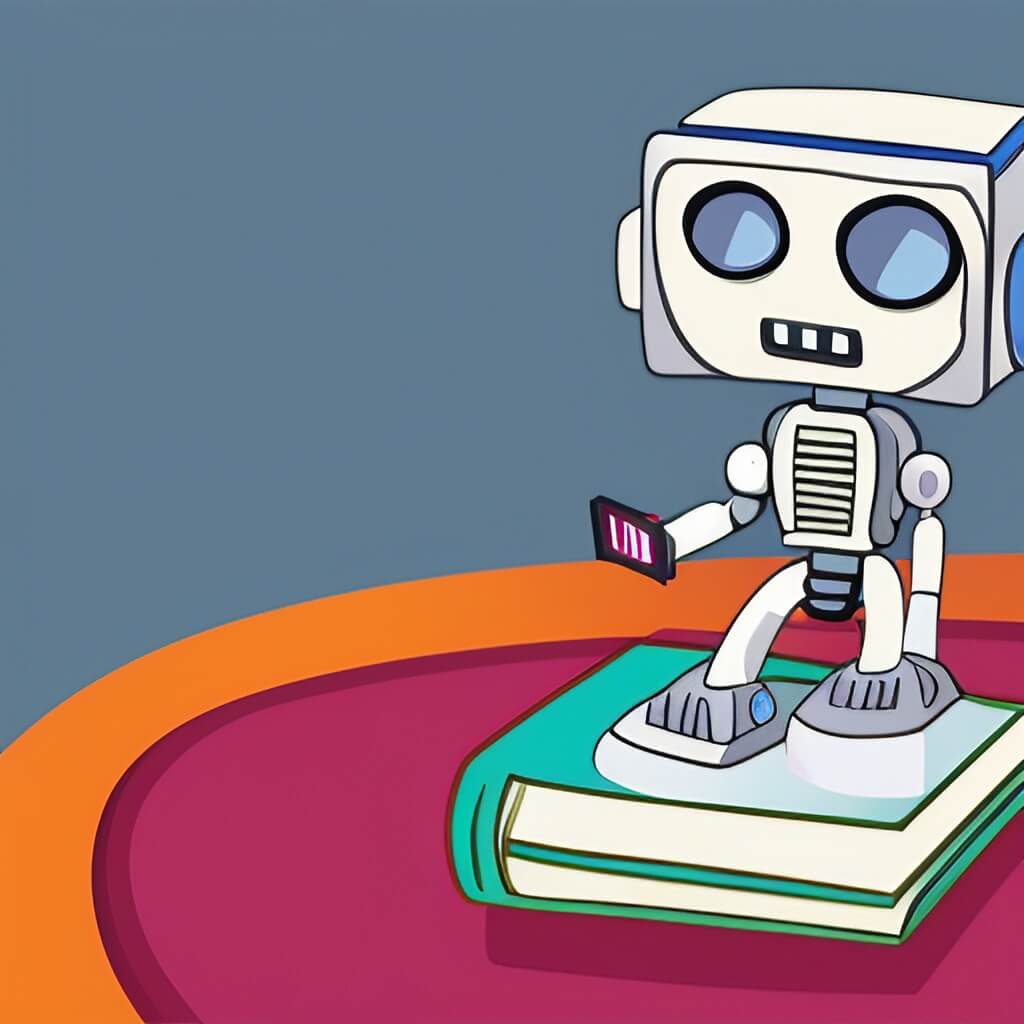
10 Best AI Copywriting Tools for 2024
Looking for the best AI copywriting tools to quickly create high-quality content? We review the top 10 AI copywriters for content marketers and copywriters.
October 9, 2024
|
Krista Doyle

The Best AI Text-to-Image Generator for the Modern Artist
AI text-to-image AI text-to-image generators are a lot of fun. But here are 5 tools that are helping modern artists work smarter, and harder.
October 6, 2024
|
Jasper Marketing

How to Create a Webinar
Creating a webinar from scratch may seem daunting, but with the right approach, your webinars can successfully educate and engage your audience.
September 30, 2024
|
Kaleigh Moore
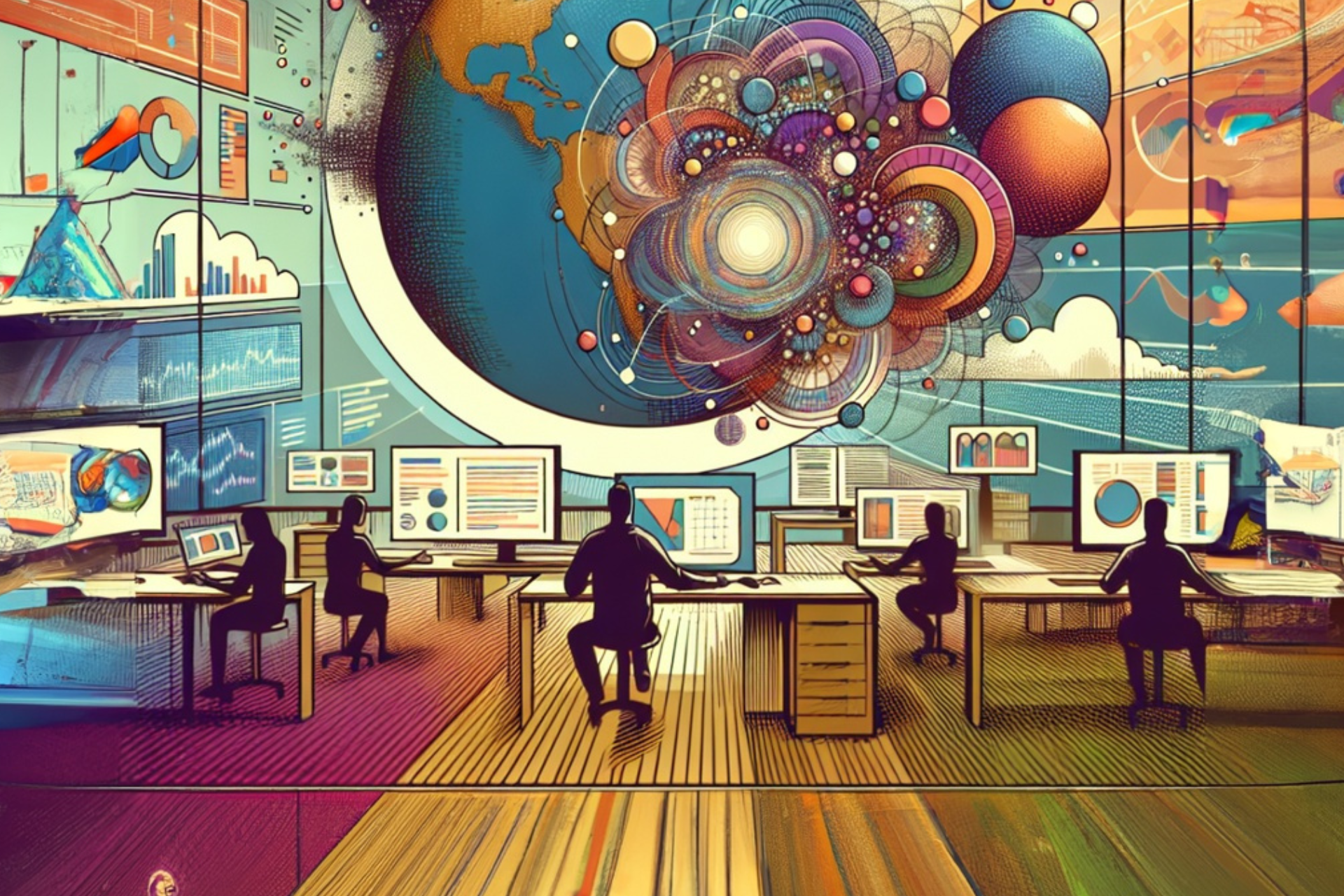
A Beginner's Guide to Content Planning: Tips and Tools
An effective content plan can ensure consistency and quality in your content creation efforts. Here's what you need to know as you dive into content planning.
September 30, 2024
|
Kaleigh Moore

Beginner's Guide to Ad Copywriting: Tips and Tricks
Understand the key elements of effective ad copy and you’ll be well on your way to crafting compelling ads that convert.
September 30, 2024
|
Kaleigh Moore
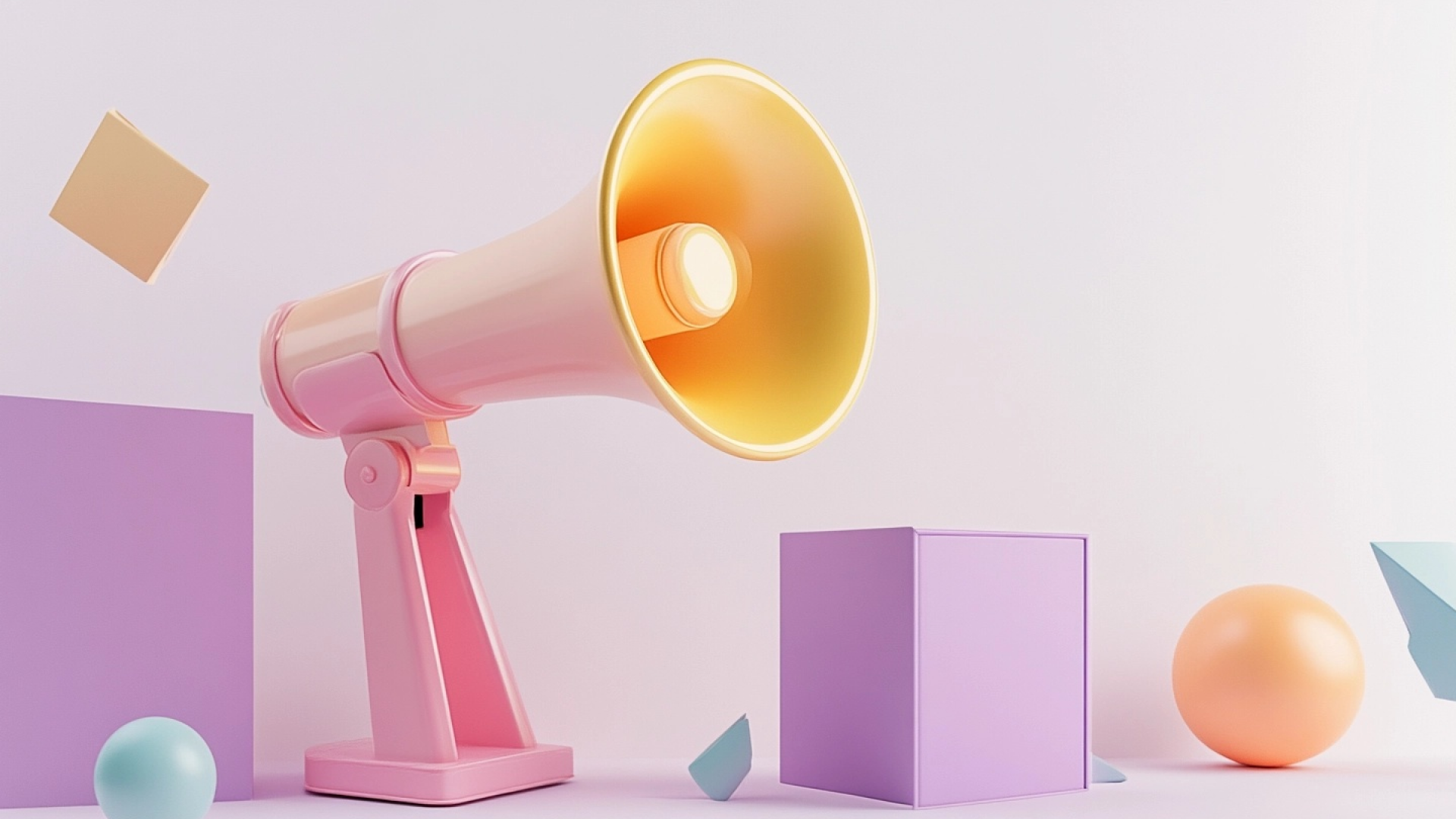
Rewriting the Playbook: 5 SaaS Companies Defining the Next Generation of Content Marketing
Here are five brands whose content programs are leading the way in the new era of content marketing.
September 30, 2024
|
Jimmy Daly
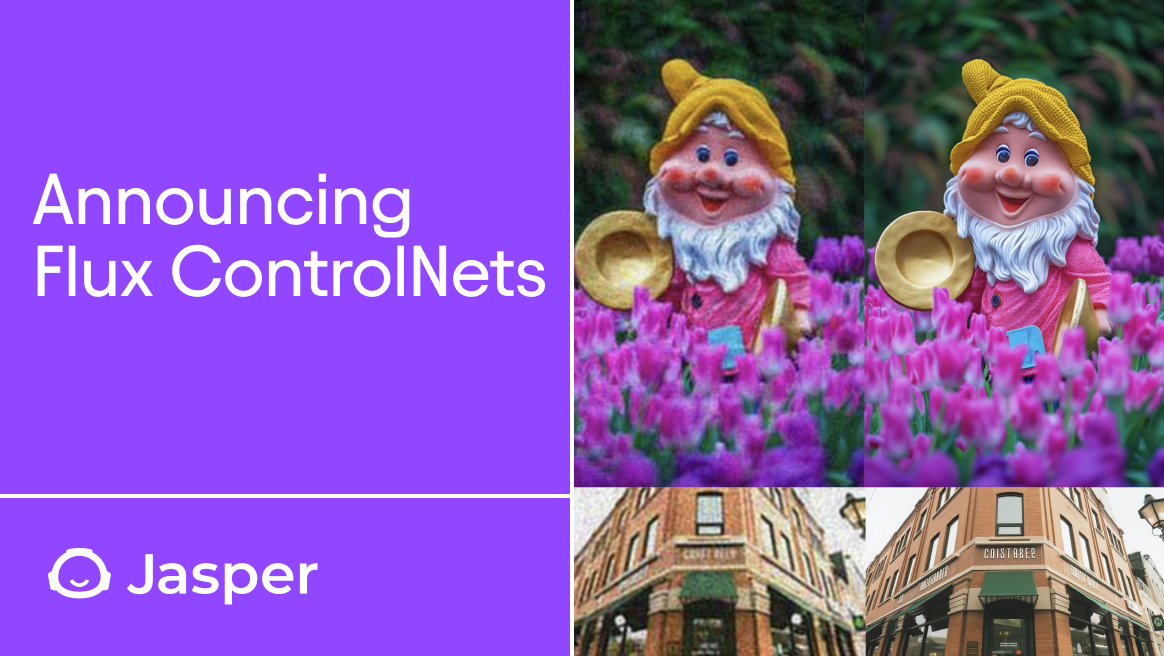
Jasper Research Releases 3 New Models to Improve Image Output for Marketers
Jasper releases three innovative new Flux ControlNets to reinforce commitment to elevating all marketing (and all marketers) with the power of AI.
September 30, 2024
|
Melody Meckfessel
.png)
Customer Marketing 101: A Comprehensive Guide for Beginners
Customer marketing encourages repeat visits, enhances the customer experience, and fosters a sense of loyalty and exclusivity.
September 25, 2024
|
Kaleigh Moore
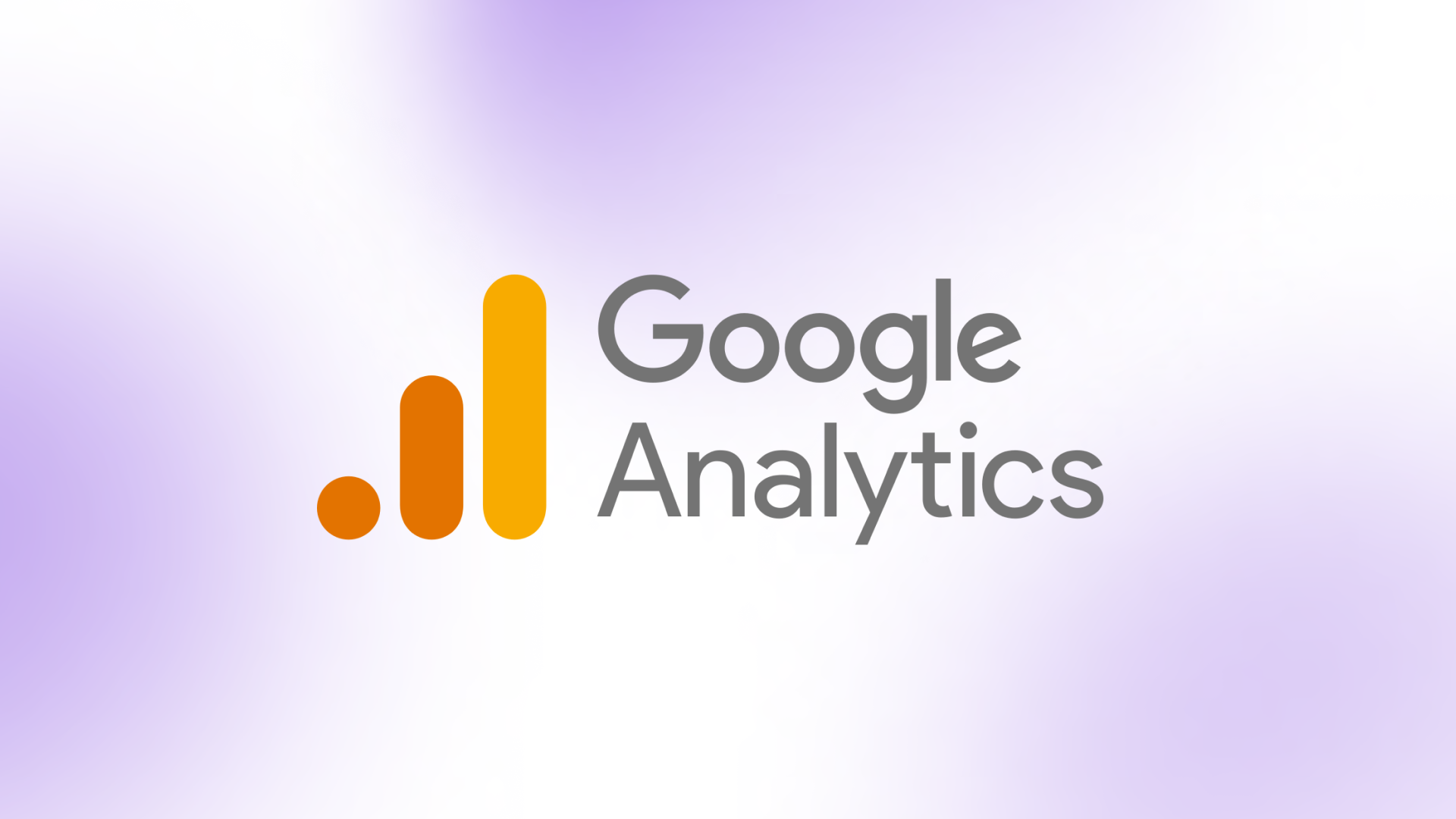
How to Use Google Analytics: A Beginner's Guide
Learn how to use Google Analytics with our comprehensive guide. Get set up, learn key features, and tips to maximize your website's performance.
September 25, 2024
|
Chris Tweten

Best Zapier Integrations for Automating Workflows
Here’s a look at the best Zapier integrations for automating and optimizing workflows for sales, content marketing, and recruitment departments across multiple
September 19, 2024
|
Amanda Laine
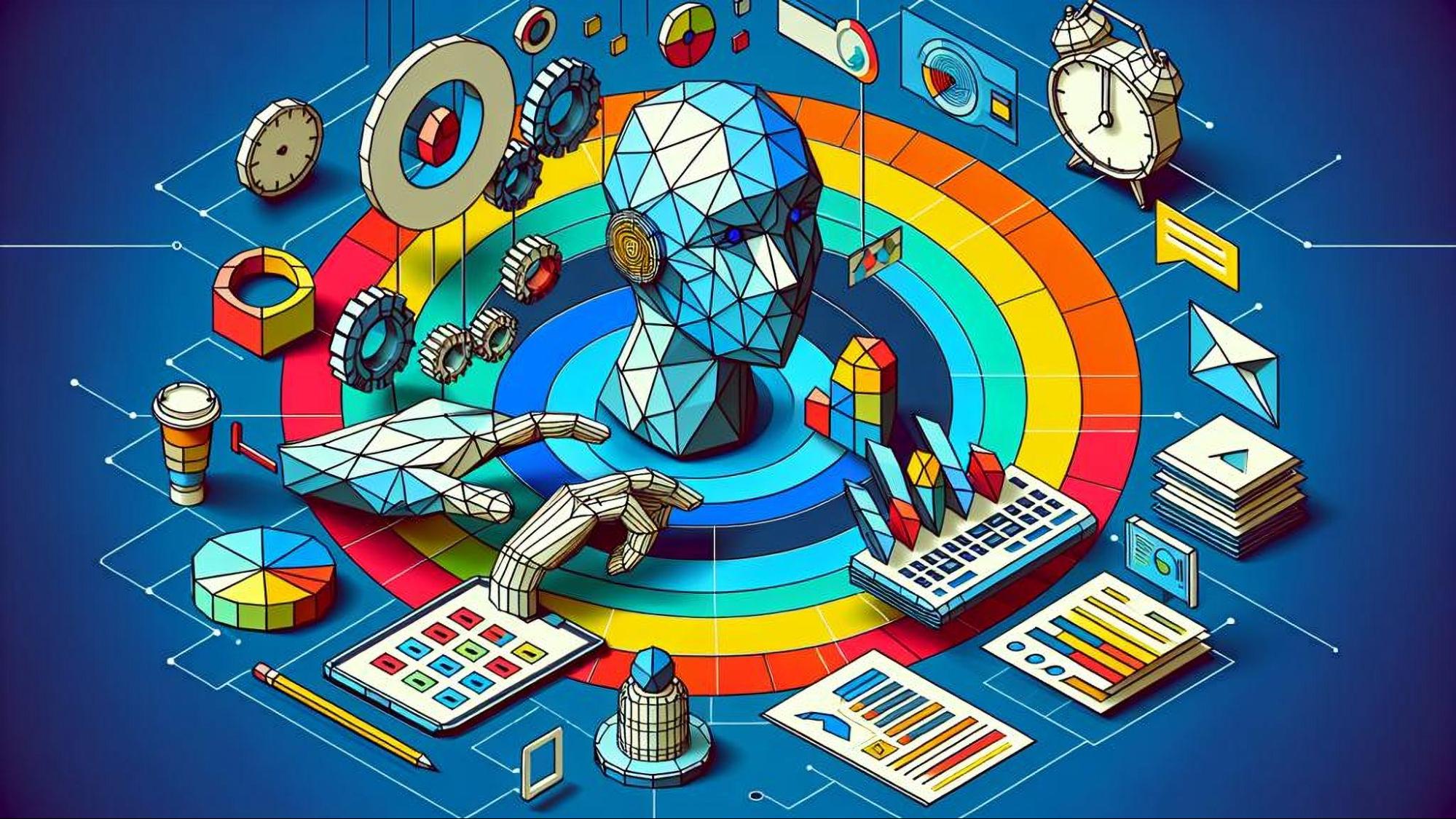
Best AI Marketing Tools of 2024: Top Picks for Every Marketer
Explore the best AI marketing tools of 2024. Find top picks, features, and benefits to elevate your marketing strategy, attract leads, and convert prospects.
September 17, 2024
|
Amanda Laine

The Best Copy.ai Alternatives for Your Content Marketing Needs
Here are three cost-effective, intuitive, and powerful Copy AI alternatives that can save you hours by automating large-scale content marketing workflows and pr
September 15, 2024
|
Amanda Laine
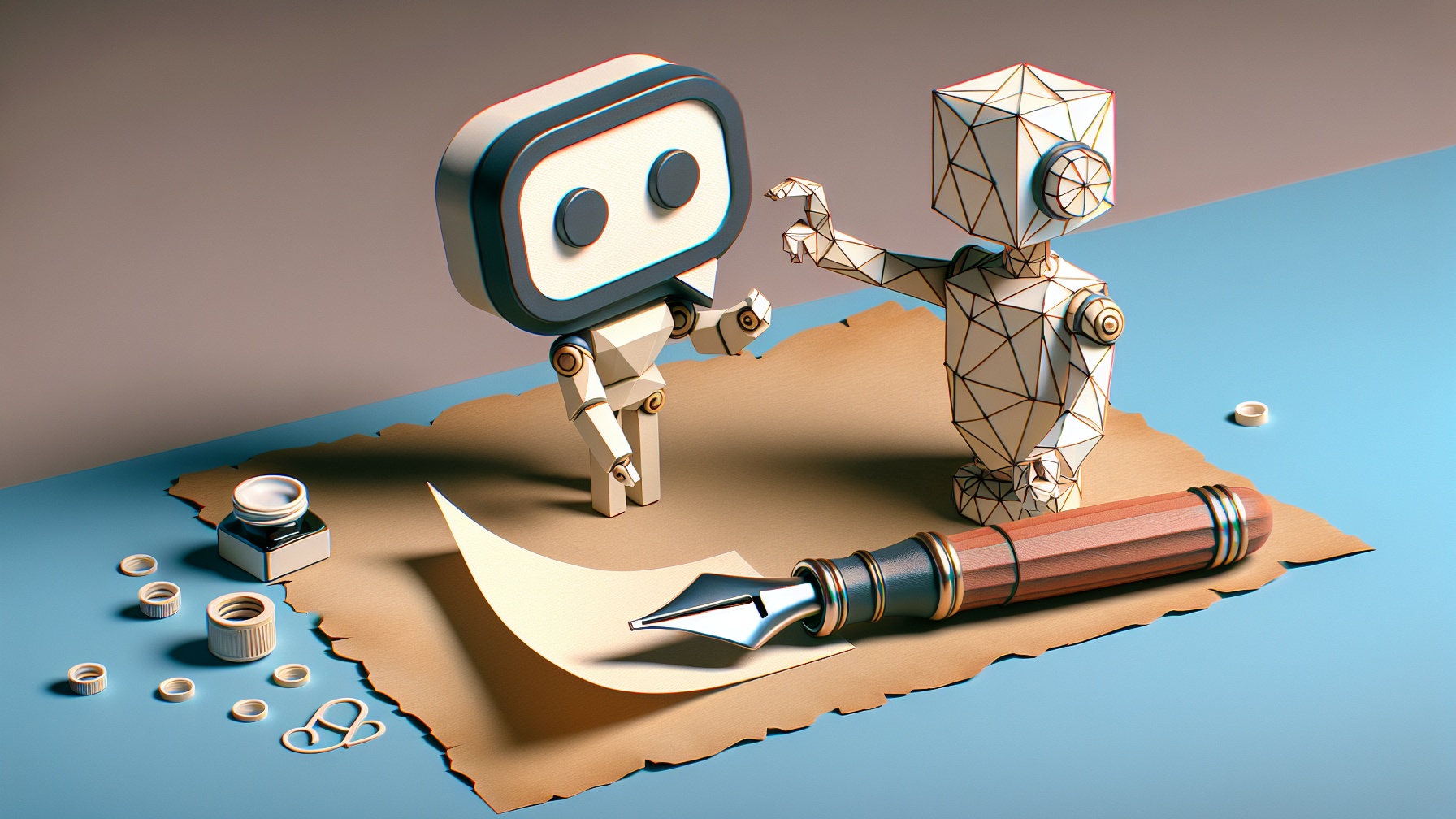
Best ChatGPT Alternatives For Content Marketing in 2024
Discover the best ChatGPT alternatives of 2024, offering unique streamlined features and revitalized capabilities for content marketing.
September 12, 2024
|
Amanda Laine
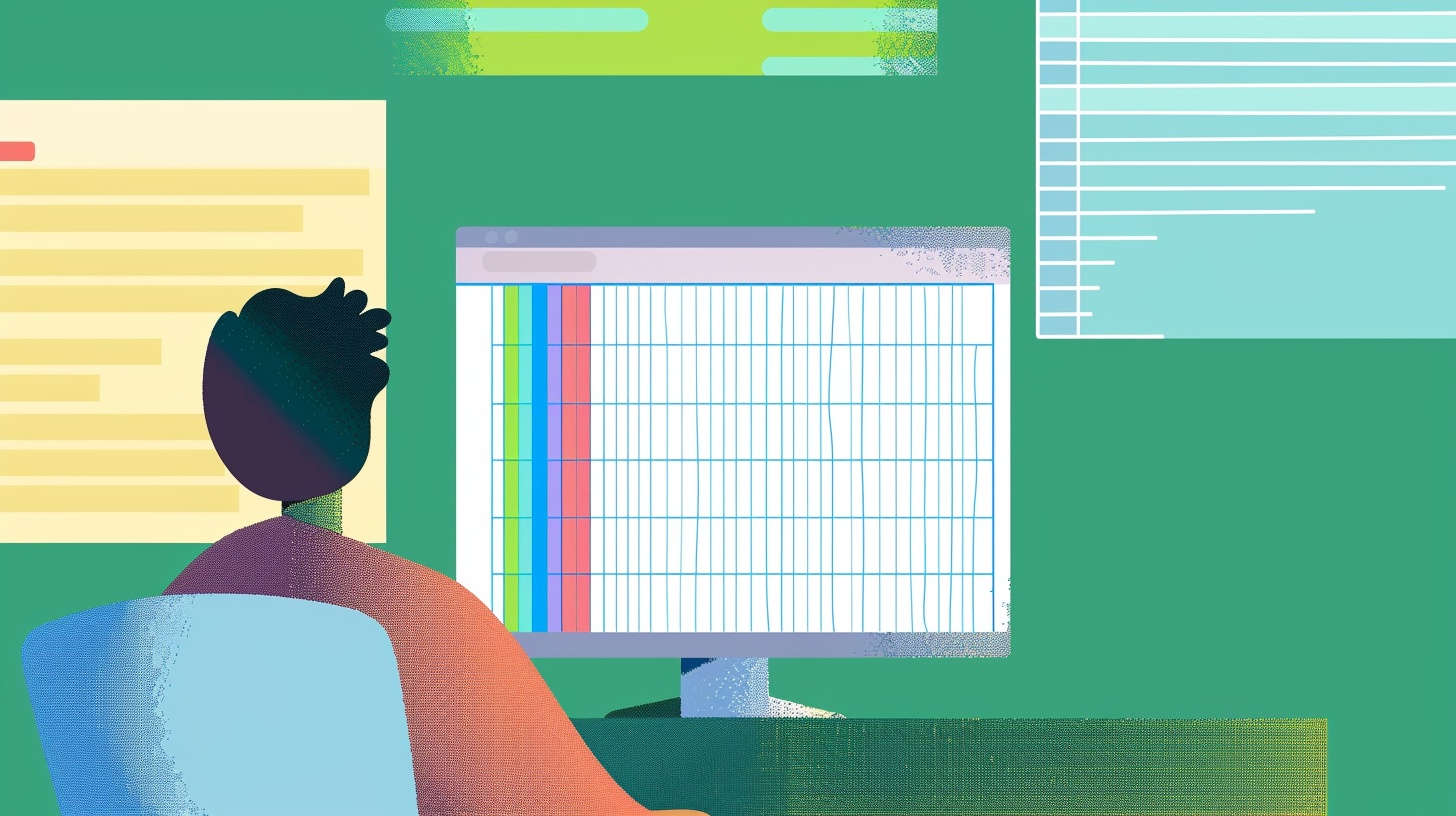
How to Use Google Sheets: A Beginner's Guide
Learn how to use Google Sheets effectively with our comprehensive guide, packed with tips, tricks, and best practices for maximizing productivity.
September 3, 2024
|
Chris Tweten
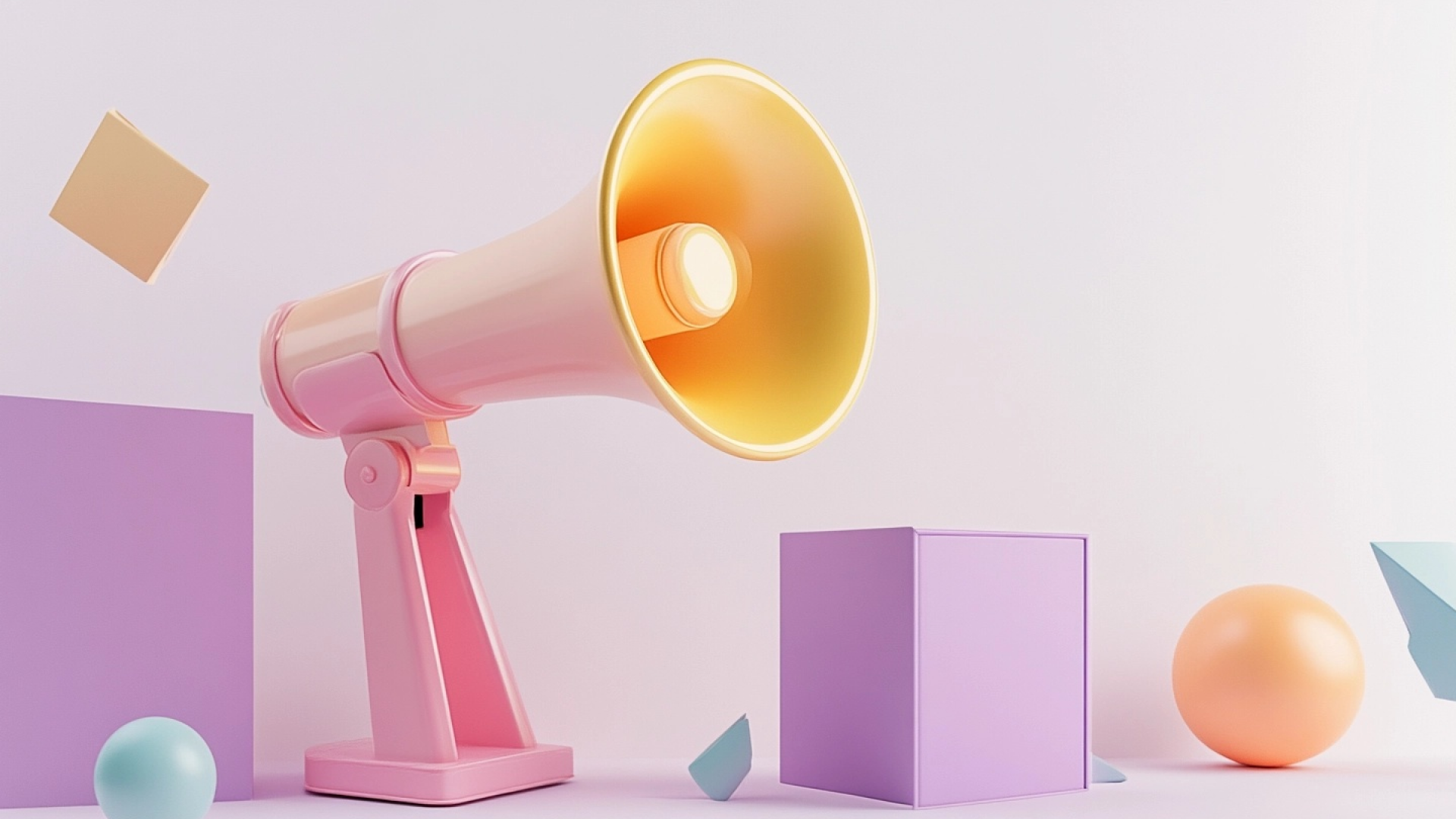
The Complete Guide to Marketing Campaigns for Beginners
A well-executed marketing campaign can elevate brand awareness, drive customer engagement, and ultimately boost sales.
September 2, 2024
|
Kaleigh Moore

How to Create Effective Blog Outlines
An effective blog outline helps organize your thoughts and ideas, enhances readability, boosts SEO, and keeps your readers engaged.
August 27, 2024
|
Kaleigh Moore
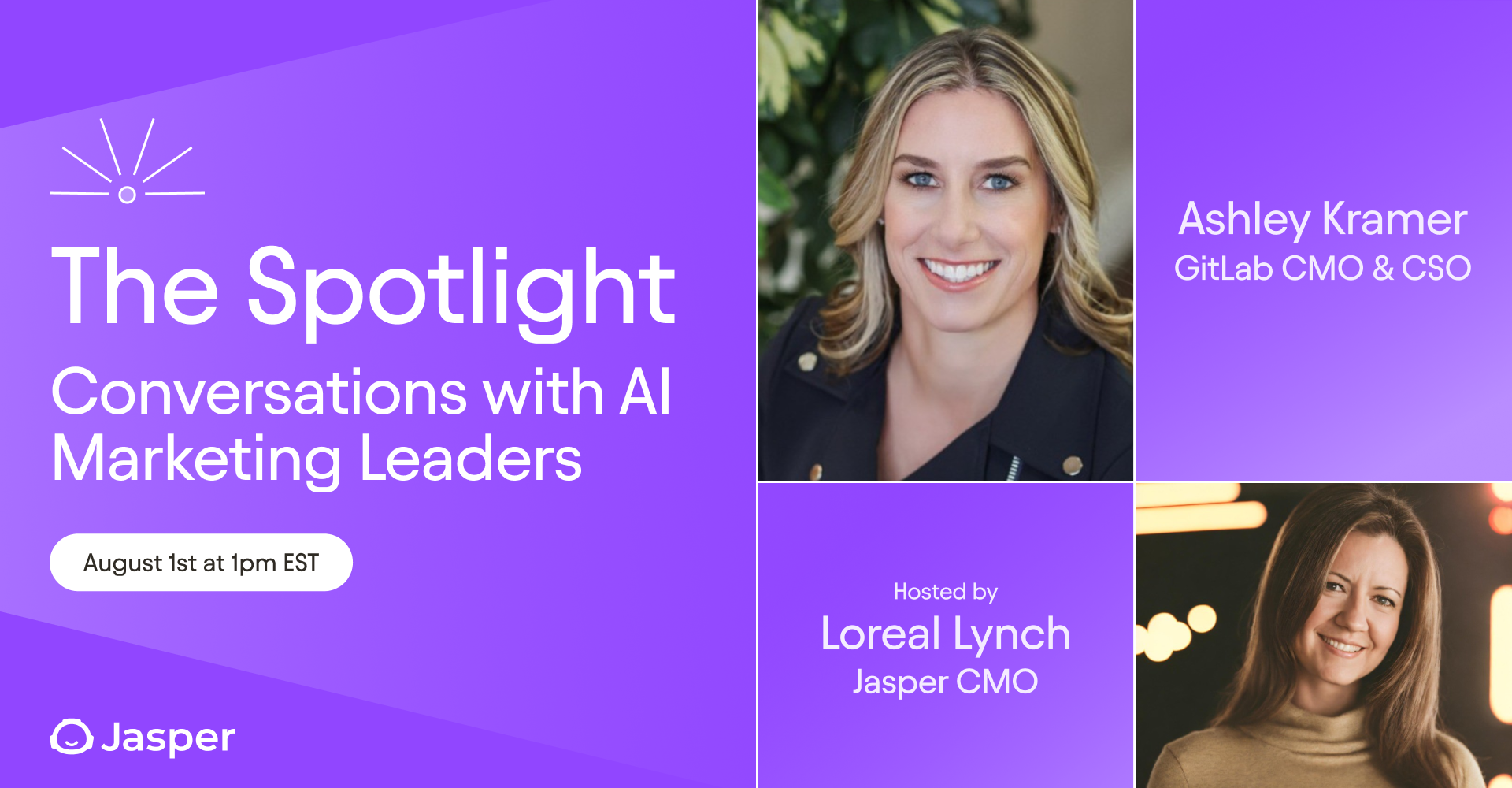
A Whole-Company Approach to AI: How GitLab is Embracing Jasper to Scale Marketing While Retaining Data security
See how Gitlab's Ashley Kramer’s top-down approach to AI implementation has made it possible to embrace the AI future.
August 15, 2024
|
Kaleigh Moore
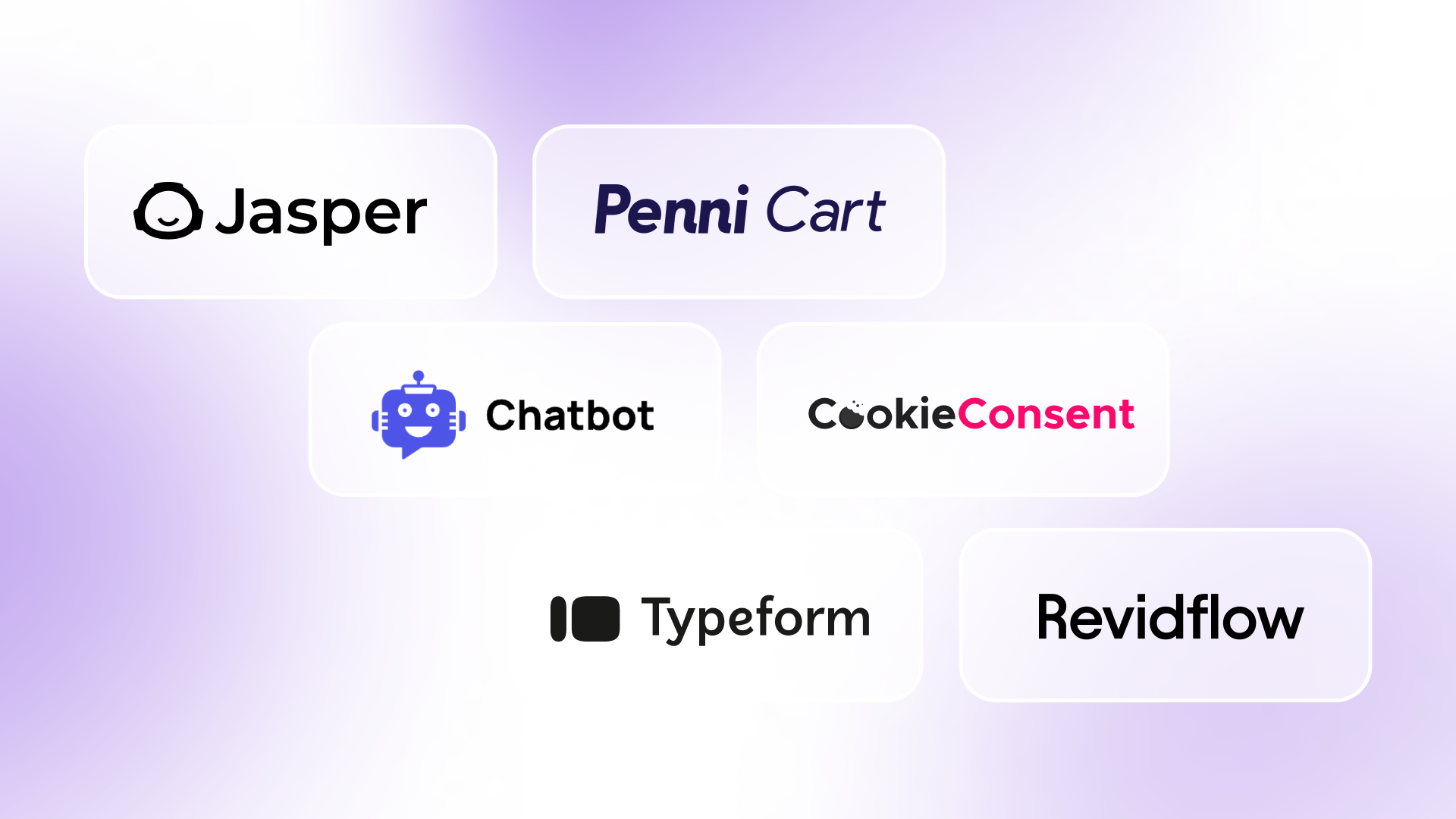
Webflow Integrations: The Essential Tools Every Website Needs
Here are 7 of the best Webflow integrations to help you improve product pages, generate reviews, auto-generate engaging website copy, and automate email marketi
July 2, 2024
|
Chris Tweten
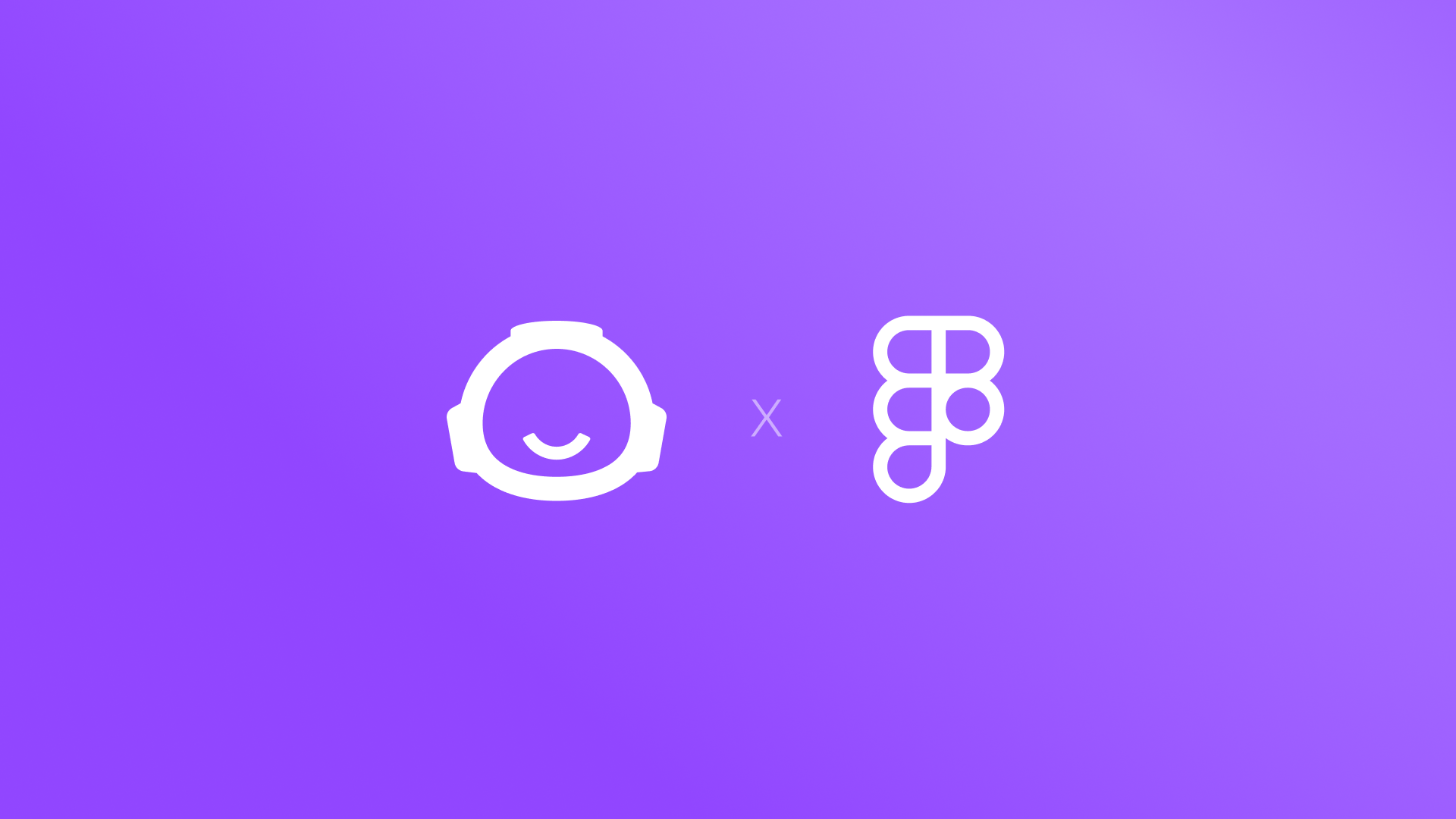
Jasper Joins Forces with Figma to Power New AI Background Removal Feature
Figma’s using Jasper to power its new background removal features. Here's what that looks like.
June 26, 2024
|
Jasper

Must-Have AI Productivity Tools for Modern Professionals
Here’s a look into the top AI productivity tools to enhance workflow and boost efficiency in 2024. Explore our curated list and find the perfect tools for your
June 18, 2024
|
Chris Tweten
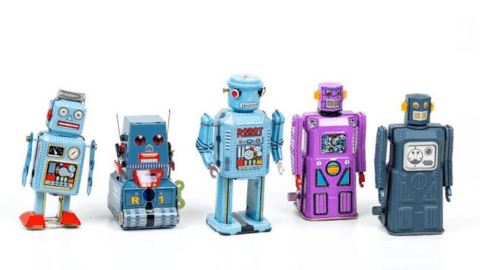
AI for Marketing: Top Tips and Tools from Marketing Experts [2024]
AI marketing: the complete guide from Jasper's in-house marketing team. Build your AI marketing strategy with these tools and tips.
June 14, 2024
|
Krista Doyle
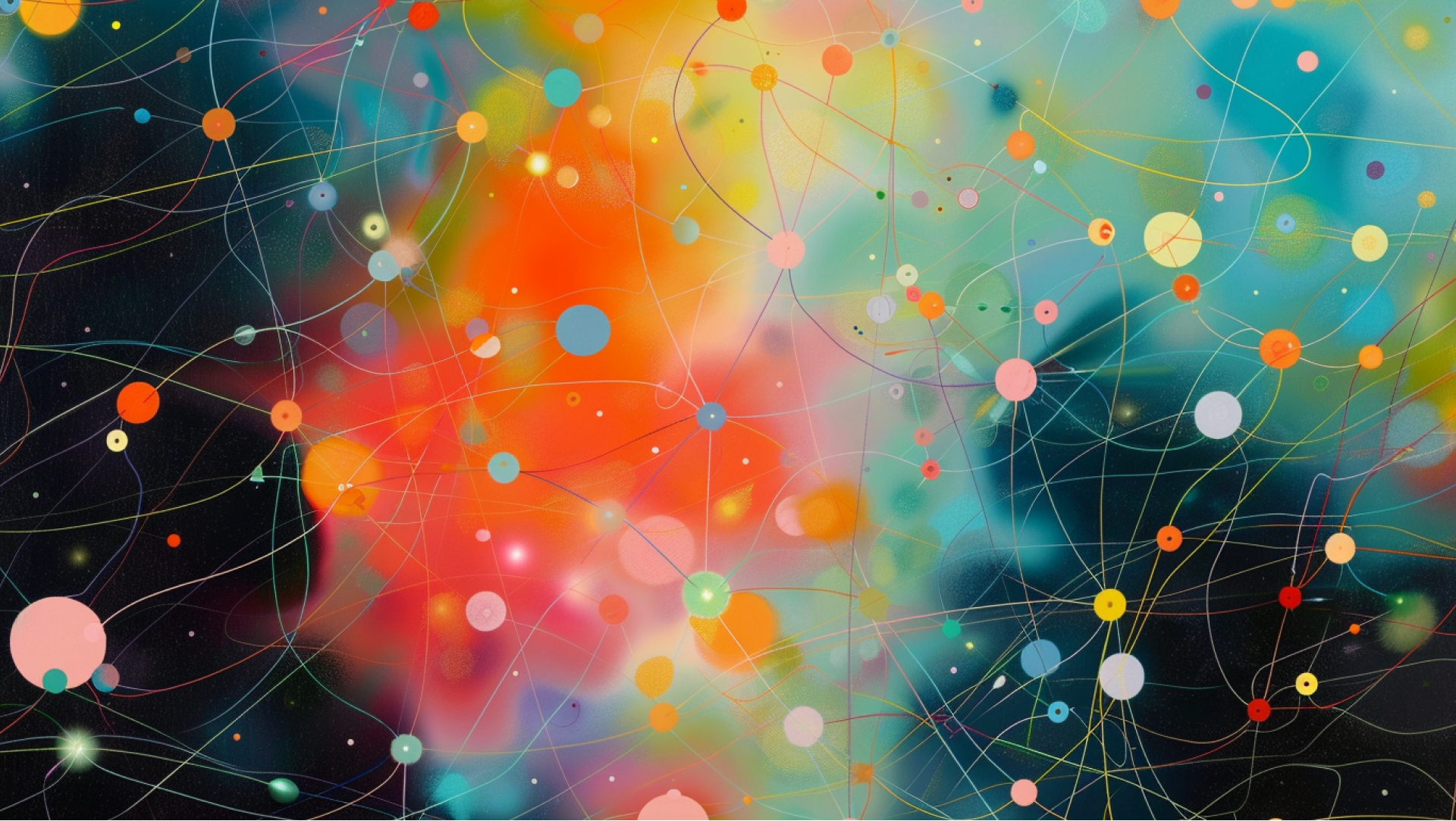
Content Distribution: A Beginner's Guide to Creating a Distribution Strategy
Let’s look at what content distribution is in 2024, and how can you get your content in front of the right people at the right time.
June 14, 2024
|
Kaleigh Moore
.png)
How to Create a Content Calendar Your Team Actually Uses
Let’s break down the content calendar creation process into easy, actionable steps and look at best practices, industry examples, and more.
June 14, 2024
|
Kaleigh Moore
.png)
How to Create an Effective Content Brief (with Examples and Templates)
Let’s look at a few of the essential elements that make for a comprehensive and actionable content brief.
June 14, 2024
|
Kaleigh Moore
.png)
How to Measure Content Marketing ROI (with Tools and Examples)
Learn how to measure content marketing ROI with these essential tips, tools, and techniques to ensure your content strategy drives results.
June 10, 2024
|
Kaleigh Moore
.png)
Flash Diffusion: Accelerating Image Generation with Diffusion Models
The latest research from Jasper signals a significant milestone for AI image generation & out-performs contributions from major players including MIT and Adobe.
June 5, 2024
|
Jasper

6 Key Tips for Writing a Marketing Brochure That Doesn't Suck
Brochures may seem like relics of a different marketing age, but don’t be fooled. Here’s how to write a brochure that sells your business (print or digital).
June 3, 2024
|
Krista Doyle

The Top 17 Affiliate Marketing Tools [2024 Update]
Do you have the right affiliate marketing tools in your arsenal to be successful? Find out as we cover 17 conversion-focused tools for 2021 and beyond!
June 3, 2024
|
Dave Rogenmoser
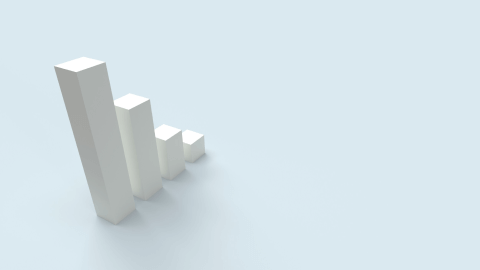
10 Apps That Write Essays For You + How to Use Them
Writing essays can be hours of long work reading and writing. To help you hand your paper in faster, we’ve compiled the top 10 apps that write essays for you.
June 3, 2024
|
Krista Doyle

5 Video Script Templates for Impactful Enterprise Marketing
Discover 5 video script templates to create impactful content that boosts engagement.
June 3, 2024
|
Jasper Marketing

The Ultimate Guide to Writing Engaging Long-Form Content
Long-form content is perfect for educating readers and establishing your position as an industry expert. Here’s how you can get started.
June 3, 2024
|
Krista Doyle

7 Steps to a Profitable Affiliate Marketing Content Strategy (with Examples)
As an affiliate marketer, you need a great affiliate marketing content strategy to succeed. Use this guide to choose the right content for your site.
June 3, 2024
|
Jasper Marketing

18 Top Blogging Tools For Strategy, Writing, SEO and More
What are the best blogging tools? Check out our list of the top 15 for everything from strategy to distribution and for everyone from beginners to pro bloggers.
June 3, 2024
|
Krista Doyle





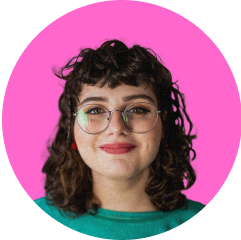
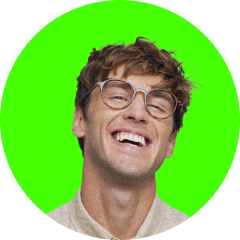
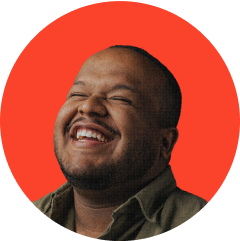